Documents
Presentation Slides
Adaptive Adversarial Cross-Entropy Loss for Sharpness-Aware Minimization
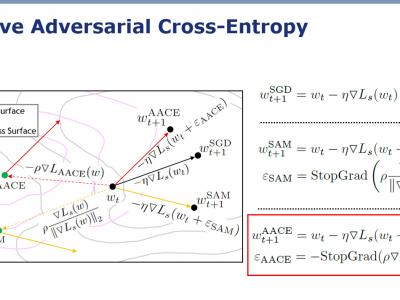
- Citation Author(s):
- Submitted by:
- TANAPAT RATCHATORN
- Last updated:
- 19 November 2024 - 5:52am
- Document Type:
- Presentation Slides
- Document Year:
- 2024
- Event:
- Presenters:
- Tanapat Ratchatorn
- Paper Code:
- MP2.L6.2
- Categories:
- Log in to post comments
Recent advancements in learning algorithms have demonstrated that the sharpness of the loss surface is an effective measure for improving the generalization gap. Building upon this concept, Sharpness-Aware Minimization (SAM) was proposed to enhance model generalization and achieved state-of-the-art performance. SAM consists of two main steps, the weight perturbation step and the weight updating step. However, the perturbation in SAM is determined by only the gradient of the training loss, or cross-entropy loss. As the model approaches a stationary point, this gradient becomes small and oscillates, leading to inconsistent perturbation directions and also has a chance of diminishing the gradient. Our research introduces an innovative approach to further enhancing model generalization. We propose the Adaptive Adversarial Cross-Entropy (AACE) loss function to replace standard cross-entropy loss for SAM's perturbation. AACE loss and its gradient uniquely increase as the model nears convergence, ensuring consistent perturbation direction and addressing the gradient diminishing issue. Additionally, a novel perturbation-generating function utilizing AACE loss without normalization is proposed, enhancing the model's exploratory capabilities in near-optimum stages. Empirical testing confirms the effectiveness of AACE, with experiments demonstrating improved performance in image classification tasks using Wide ResNet and PyramidNet across various datasets. The reproduction code is available online: http://www.vip.sc.e.titech.ac.jp/proj/AACE