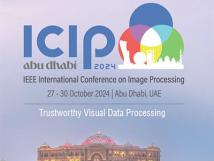
IEEE ICIP 2024 - The International Conference on Image Processing (ICIP), sponsored by the IEEE Signal Processing Society, is the premier forum for the presentation of technological advances and research results in the fields of theoretical, experimental, and applied image and video processing. ICIP has been held annually since 1994, brings together leading engineers and scientists in image and video processing from around the world. Visit website.
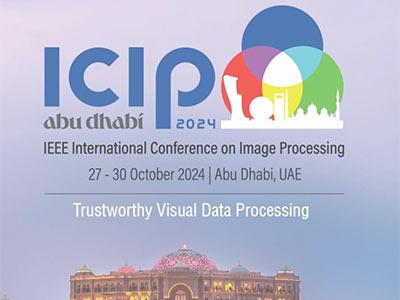
- Read more about Fourier Ptychography Microscopy With Integrated Positional Misalignment Correction
- Log in to post comments
Fourier Ptychography Microscopy enables reconstructing both intensity and phase high-resolution wide-field images from multiple captures under varying illumination directions. The capture process is classically modeled using a neural network. The reconstructed object is iteratively optimized by gradient descent so the network output matches the captures. Although, this process hinges on a precise estimation of the system geometry.
- Categories:
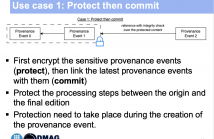
The surge of media consumption on the Internet reflects how individuals engage with information, entertainment and communication. In parallel, the advancement of generative AI tools facilitates the creation of abundant content that is nearly indistinguishable from authentic content. To address aspects of misinformation, focus is shifted towards the secure and immutable annotation of provenance information. Although such frameworks aim to establish trust in media consumption, they raise privacy concerns, as provenance data may conceal identifiable information for individuals and locations.
- Categories:
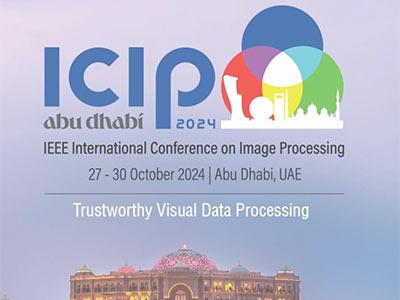
- Read more about Increasing Trust in Image Analysis by Detecting Trellis Quantization in JPEG Images - ICIP'24 Slides
- Log in to post comments
JPEG image forensics investigates the authenticity and origin of compressed images. Many established methods rely on assumptions about the statistical distribution of quantized discrete cosine transform coefficients. However, JPEG implementations that use trellis quantization, such as mozjpeg, produce images that challenge these assumptions. In this study, we demonstrate that artifacts resulting from trellis quantization can compromise the reliability of established forensic methods and cause false alarms for innocuous images.
- Categories:
- Read more about Transforming Tabular Data For Multi-modality: Enhancing Breast Cancer Metastasis Prediction Through Data Conversion
- Log in to post comments
Breast cancer metastasis prediction plays a key role in
clinical decision-making and secondary analysis. Traditionally,
metastasis classification models have been developed
using structured tabular clinical data, but these approaches
may result in data loss and lack of contextual information. A
multi-modal approach is presented in this article for predicting
breast cancer metastasis by converting structured clinical
data into unstructured text, which provides more contextual
information, and then converting that text into histopathology
- Categories:
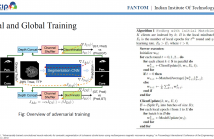
- Read more about FANTOM: Federated Adversarial Network for Training Multi-sequence Magnetic Resonance Imaging in Semantic Segmentation
- Log in to post comments
Ischemic stroke lesions (ISL) segmentation aids clinicians in the diagnosis of stroke in acute care units. But, a generalized segmentation model requires data from various patients. However considering the data privacy, the patient's data is not available for centralized training. The Federated Learning (FL) framework overcomes this, but in FL, semantic segmentation is challenging due to the complex model, adversarial training, and non-independent and identically distributed dataset.
- Categories:
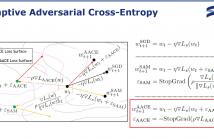
- Read more about Adaptive Adversarial Cross-Entropy Loss for Sharpness-Aware Minimization
- Log in to post comments
Recent advancements in learning algorithms have demonstrated that the sharpness of the loss surface is an effective measure for improving the generalization gap. Building upon this concept, Sharpness-Aware Minimization (SAM) was proposed to enhance model generalization and achieved state-of-the-art performance. SAM consists of two main steps, the weight perturbation step and the weight updating step. However, the perturbation in SAM is determined by only the gradient of the training loss, or cross-entropy loss.
- Categories:
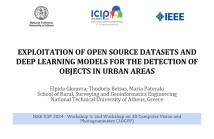
- Read more about EXPLOITATION OF OPEN SOURCE DATASETS AND DEEP LEARNING MODELS FOR THE DETECTION OF OBJECTS IN URBAN AREAS
- Log in to post comments
In this work we utilize different open-source datasets and deep learning models for detecting objects from image data captured by a mobile mapping system integrating the multi-camera Ladybug 5+ in an urban area. In our experiments
we exploit sets of pre-trained models and models trained via transfer learning techniques with available open source datasets for object detection, semantic-, instance-, and panoptic segmentation. Tests with the trained models are performed with image data from the Ladybug 5+ camera.
- Categories:
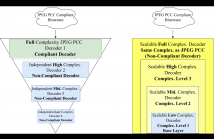
- Read more about Learning-based Point Cloud Decoding With Independent and Scalable Reduced Complexity
- 1 comment
- Log in to post comments
Point Clouds (PCs) have gained significant attention due to their usage in diverse application domains, notably virtual and augmented reality. While PCs excel in providing detailed 3D visualization, this typically requires millions of points which must be efficiently coded for real-world deployment, notably storage and streaming. Recently, learning-based coding solutions have been adopted, notably in the JPEG Pleno Point Coding (PCC) standard, which uses a coding model with millions of model parameters.
- Categories:
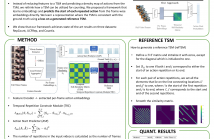
- Read more about Rethinking temporal self-similarity for repetitive action counting
- Log in to post comments
Counting repetitive actions in long untrimmed videos is a challenging task that has many applications such as rehabilitation. State-of-the-art methods predict action counts by first generating a temporal self-similarity matrix (TSM) from the sampled frames and then feeding the matrix to a predictor network. The self-similarity matrix, however, is not an optimal input to a network since it discards too much information from the frame-wise embeddings.
- Categories:
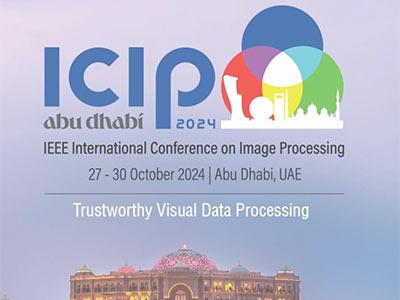
- Read more about Fast Unsupervised Tensor Restoration via Low-rank Deconvolution
- Log in to post comments
Low-rank Deconvolution (LRD) has appeared as a new multi-dimensional representation model that enjoys important efficiency and flexibility properties. In this work we ask ourselves if this analytical model can compete against Deep Learning (DL) frameworks like Deep Image Prior (DIP) or Blind-Spot Networks (BSN) and other classical methods in the task of signal restoration. More specifically, we propose to extend LRD with differential regularization.
- Categories: