Documents
Poster
ADAPTIVE ATTENTION GRAPH CAPSULE NETWORK
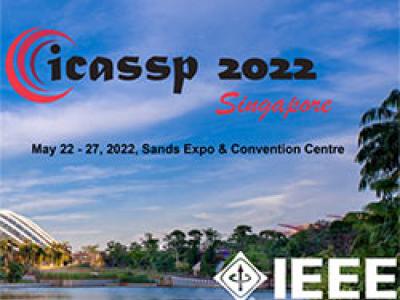
- Citation Author(s):
- Submitted by:
- Xiangping Zheng
- Last updated:
- 5 May 2022 - 5:23am
- Document Type:
- Poster
- Document Year:
- 2022
- Event:
- Presenters:
- Xiangping Zheng
- Paper Code:
- MLSP-17.5
- Categories:
- Log in to post comments
From the perspective of the spatial domain, Graph Convolutional Network (GCN) is essentially a process of iteratively aggregating neighbor nodes. However, the existing GCNs using simple average or sum aggregation may neglect the characteristics of each node and the topology between nodes, resulting in a large amount of early-stage information lost during the graph convolution step. To tackle the above challenge, we innovatively propose an adaptive attention graph capsule network, named AA-GCN, for graph classification. We explore various propagation mechanisms of graphs and present an attention mechanism combined with graph propagation and capsules to generate capsule nodes, preserving the spatial topology between nodes. We also propose a graph adaptive attention mechanism to investigate the context information in different global GCN layers, so as to effectively improve the next dynamic routing connection and the final graph classification. Experiments show that our proposed algorithm achieves either state-of-the-art or competitive results across all the datasets.