Documents
Poster
ADAPTIVE CONFIDENCE MULTI-VIEW HASHING FOR MULTIMEDIA RETRIEVAL
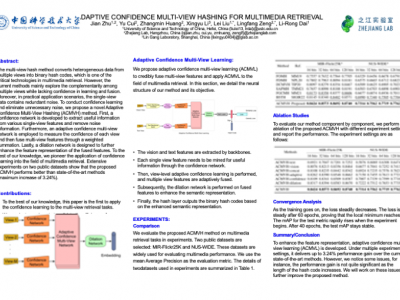
- Citation Author(s):
- Submitted by:
- Jian Zhu
- Last updated:
- 11 April 2024 - 7:23pm
- Document Type:
- Poster
- Categories:
- Log in to post comments
The multi-view hash method converts heterogeneous data from multiple views into binary hash codes, which is one of the critical technologies in multimedia retrieval. However, the current methods mainly explore the complementarity among multiple views while lacking confidence in learning and fusion. Moreover, in practical application scenarios, the single-view data contains redundant noise. To conduct confidence learning and eliminate unnecessary noise, we propose a novel Adaptive Confidence Multi-View Hashing (ACMVH) method. First, a confidence network is developed to extract useful information from various single-view features and remove noise information. Furthermore, an adaptive confidence multi-view network is employed to measure the confidence of each view and then fuse multi-view features through a weighted summation. Lastly, a dilation network is designed to further enhance the feature representation of the fused features. To the best of our knowledge, we pioneer the application of confidence learning to the field of multimedia retrieval. Extensive experiments on two public datasets show that the proposed ACMVH performs better than state-of-the-art methods (maximum increase of 3.24%). The source code is available at https://github.com/HackerHyper/ACMVH.