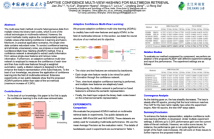
- Read more about ADAPTIVE CONFIDENCE MULTI-VIEW HASHING FOR MULTIMEDIA RETRIEVAL
- Log in to post comments
The multi-view hash method converts heterogeneous data from multiple views into binary hash codes, which is one of the critical technologies in multimedia retrieval. However, the current methods mainly explore the complementarity among multiple views while lacking confidence in learning and fusion. Moreover, in practical application scenarios, the single-view data contains redundant noise. To conduct confidence learning and eliminate unnecessary noise, we propose a novel Adaptive Confidence Multi-View Hashing (ACMVH) method.
- Categories:
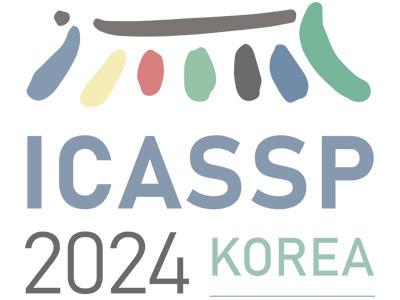
- Read more about Video-Language Graph Convolutional Network for Human Action Recognition
- Log in to post comments
Transferring visual language models (VLMs) from the image domain to the video domain has recently yielded great success on human action recognition tasks. However, standard recognition paradigms overlook fine-grained action parsing knowledge that could enhance the recognition accuracy. In this paper, we propose a novel method that leverages both coarse-grained and fine-grained knowledge to recognize human actions in videos. Our method consists of a video-language graph convolutional network that integrates and fuses multi-modal knowledge in a progressive manner.
- Categories:
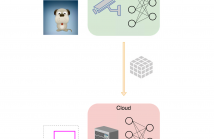
- Read more about Edge-Cloud Collaborative Multimedia Analysis
- Log in to post comments
Our world is at the beginning of the technological revolution that promises to transform the way we work, travel, learn, and live, through Artificial Intelligence (AI). While AI models have been making tremendous progress in research labs and overtaking scientific literature in many fields, efforts are now being made to take these models out of the lab and create products around them, which could compete with established technologies in terms of cost, reliability, and user trust, as well as enable new, previously unimagined applications.
- Categories:
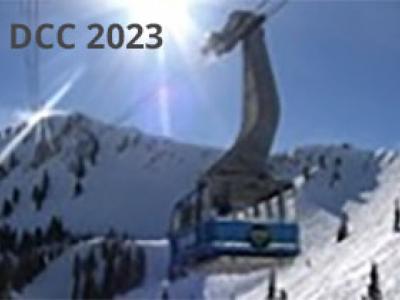
- Categories:
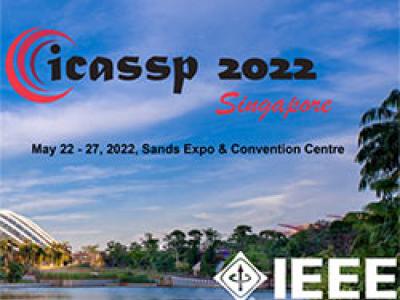
- Read more about IVMSP-18.3: IMAGE-TO-VIDEO RE-IDENTIFICATION VIA MUTUAL DISCRIMINATIVE KNOWLEDGE TRANSFER
- 1 comment
- Log in to post comments
The gap in representations between image and video makes Image-to-Video Re-identification (I2V Re-ID) challenging, and recent works formulate this problem as a knowledge distillation (KD) process. In this paper, we propose a mutual discriminative knowledge distillation framework to transfer a video-based richer representation to an image based representation more effectively. Specifically, we propose the triplet contrast loss (TCL), a novel loss designed for KD.
- Categories:
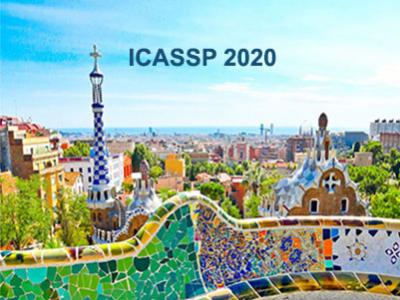
We propose a computational framework for ranking images (group photos in particular) taken at the same event within a short time span. The ranking is expected to correspond with human perception of overall appeal of the images. We hypothesize and provide evidence through subjective analysis that the factors that appeal to humans are its emotional content, aesthetics and image quality. We propose a network which is an ensemble of three information channels, each predicting a score corresponding to one of the three visual appeal factors.
- Categories:
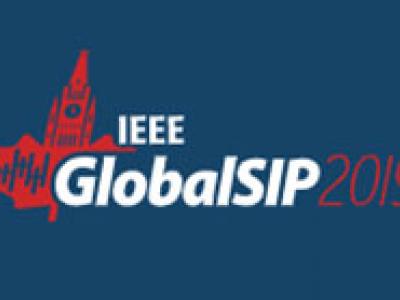
- Read more about Super-Resolution for Imagery Enhancement Using Variational Quantum Eigensolver
- Log in to post comments
Super-Resolution (SR) is a technique that has been exhaustively exploited and incorporates strategic aspects to image processing. As quantum computers gradually evolve and provide unconditional proof of computational advantage at solving intractable problems over their classical counterparts, quantum computing emerges with the compelling prospect to offer exponential speedup to process computationally expensive operations, such as the ones verified in SR imaging.
- Categories:
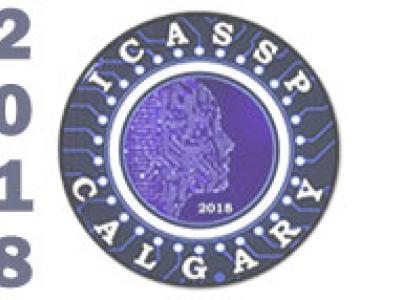
- Read more about A DEEP REINFORCEMENT LEARNING FRAMEWORK FOR IDENTIFYING FUNNY SCENES IN MOVIES
- Log in to post comments
This paper presents a novel deep Reinforcement Learning (RL)framework for classifying movie scenes based on affect using the face images detected in the video stream as input. Extracting affective information from the video is a challenging task modulating complex visual and temporal representations intertwined with the complex aspects of human perception and information integration. This also makes it difficult to collect a large annotated corpus restricting the use of supervised learning methods.
- Categories:
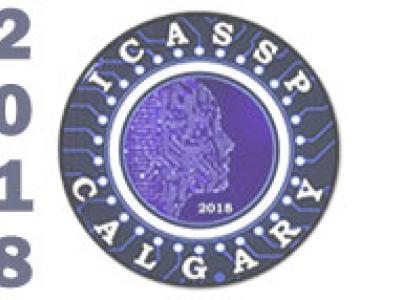
This paper presents a general framework for model-based 3D face reconstruction from a single image, which can incorporate mature face alignment methods and utilize their properties. In the proposed framework, the final model parameters, i.e., mostly including pose, identity and expression, are achieved by estimating updating the face landmarks and 3D face model parameter alternately. In addition, we propose the parameter augmented regression method (PARM) as an novel derivation of the framework.
- Categories:
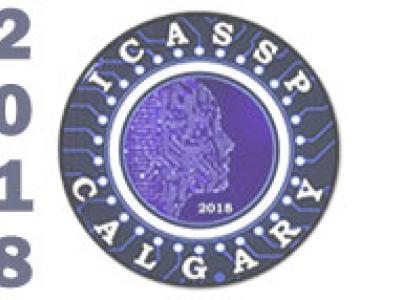
- Read more about CORRELATION-BASED FACE DETECTION FOR RECOGNIZING FACES IN VIDEOS
- Log in to post comments
- Categories: