Documents
Poster
Adaptive speech emotion representation learning based on dynamic graph
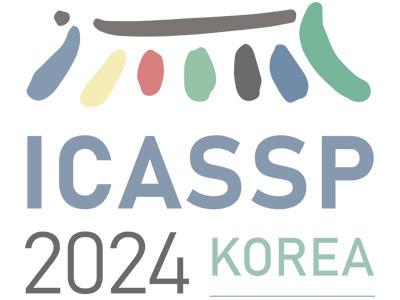
- DOI:
- 10.60864/22r3-j902
- Citation Author(s):
- Submitted by:
- yingxue gao
- Last updated:
- 6 June 2024 - 10:32am
- Document Type:
- Poster
- Document Year:
- 2024
- Event:
- Presenters:
- Yingxue Gao
- Paper Code:
- SLP-P25.5
- Categories:
- Log in to post comments
Graph representation learning has become a hot research topic due to its powerful nonlinear fitting capability in extracting representative node embeddings. However, for sequential data such as speech signals, most traditional methods merely focus on the static graph created within a sequence, and largely overlook the intrinsic evolving patterns of these data. This may reduce the efficiency of graph representation learning for sequential data. For this reason, we propose an adaptive graph representation learning method based on dynamically evolved graphs, which are consecutively constructed on a series of subsequences segmented by a sliding window. In doing this, it is better to capture local and global context information within a long sequence. Moreover, we introduce a weighted approach to update the node representation rather than the conventional average one, where the weights are calculated by a novel matrix computation based on the degree of neighboring nodes. Finally, we construct a learnable graph convolutional layer that combines the graph structure loss and classification loss to optimize the graph structure. To verify the effectiveness of the proposed method, we conducted experiments for speech emotion recognition on the IEMOCAP and RAVDESS datasets. Experimental results show that the proposed method outperforms the latest (non-)graph-based models.
Comments
Your reading and suggestions
Your reading and suggestions are welcome!