Documents
Presentation Slides
ADVANCING RNN TRANSDUCER TECHNOLOGY FOR SPEECH RECOGNITION
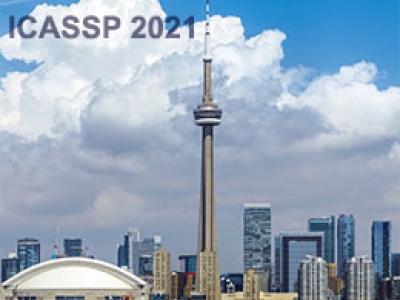
- Citation Author(s):
- Submitted by:
- George Saon
- Last updated:
- 22 June 2021 - 9:32am
- Document Type:
- Presentation Slides
- Document Year:
- 2021
- Event:
- Presenters:
- George Saon
- Paper Code:
- SPE-2.1
- Categories:
- Log in to post comments
We investigate a set of techniques for RNN Transducers (RNN-Ts) that were instrumental in lowering the word error rate on three different tasks (Switchboard 300 hours, conversational Spanish 780 hours and conversational Italian 900 hours). The techniques pertain to architectural changes, speaker adaptation, language model fusion, model combination and general training recipe. First, we introduce a novel multiplicative integration of the encoder and prediction network vectors in the joint network (as opposed to additive). Second, we discuss the applicability of i-vector speaker adaptation to RNNTs in conjunction with data perturbation. Third, we explore the effectiveness of the recently proposed density ratio language model fusion for these tasks. Last but not least, we describe the other components of our training recipe and their effect on recognition performance. We report a 5.9% and 12.5% word error rate on the Switchboard and CallHome test sets of the NIST Hub5 2000 evaluation and a 12.7% WER on the Mozilla CommonVoice Italian test set.