Documents
Presentation Slides
Analysis on Compressed Domain: A Multi-Task Learning Approach
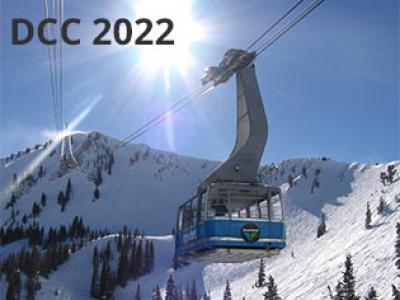
- Citation Author(s):
- Submitted by:
- Yuefeng Zhang
- Last updated:
- 4 March 2022 - 11:07am
- Document Type:
- Presentation Slides
- Document Year:
- 2022
- Event:
- Presenters:
- Yuefeng Zhang
- Paper Code:
- 184
- Categories:
- Log in to post comments
Image compression approaches based on deep learning have achieved remarkable success.
Existing studies mainly focus on human vision and machine analysis tasks taking reconstructed images as input.
However, those methods need images to be decoded before performing downstream visual tasks, which motivates us to explore how to directly conduct visual analysis using the compressed data without decoding. In this paper, we propose a multi-task learning model which can directly obtain semantic information from the compressed visual data. Specifically, a task-agnostic learning-based compression model is proposed, which effectively supports various compressed domain-based analytical tasks meanwhile reserves outstanding reconstructed perceptual quality compared with traditional and learning-based codecs. To obtain the extremely compacted data representation with essential semantic information, we take the help of the generative model on decoder part. Then, we propose a multi-task learning model which can directly obtain semantic information from the compressed visual data. In addition, joint optimization strategy is adopted to achieve the best balance point among compression efficiency, reconstructed image quality, and the downstream visual tasks' performance. Experimental results verify that our proposed compressed domain-based multi-task analysis model outperforms the reconstructed image-based method on transmission efficiency, saving more than ten times of bit-rate consumption while preserving comparable visual analysis precision (i.e., classification and segmentation tasks) when compared with RGB image input models, which is evaluated on the CelebA-HQ dataset.
Comments
Welcome to contact us if you have any questions & suggestions!
Nice to be here!!!
Follow up!
Please find more details and deeper ideas in this extended paper: https://arxiv.org/abs/2304.06896
Thank you for your attention : )