Documents
Poster
Anomaly Detection in Raw Audio Using Deep Autoregressive Networks
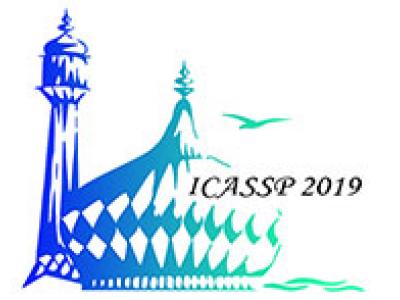
- Citation Author(s):
- Submitted by:
- Ellen Rushe
- Last updated:
- 9 May 2019 - 11:12am
- Document Type:
- Poster
- Document Year:
- 2019
- Event:
- Presenters:
- Ellen Rushe
- Paper Code:
- 4921
- Categories:
- Log in to post comments
Anomaly detection involves the recognition of patterns outside of what is considered normal, given a certain set of input data. This presents a unique set of challenges for machine learning, particularly if we assume a semi-supervised scenario in which anomalous patterns are unavailable at training time meaning algorithms must rely on non-anomalous data alone. Anomaly detection in time series adds an additional level of complexity given the contextual nature of anomalies. For time series modelling, autoregressive deep learning architectures such as WaveNet have proven to be powerful generative models, specifically in the field of speech synthesis. In this paper, we propose to extend the use of this type of architecture to anomaly detection in raw audio. In experiments using multiple audio datasets we compare the performance of this approach to a baseline autoencoder model and show superior performance in almost all cases