Documents
Poster
Artificial Bandwidth Extension of Narrowband Speech Using Generative Adversarial Networks
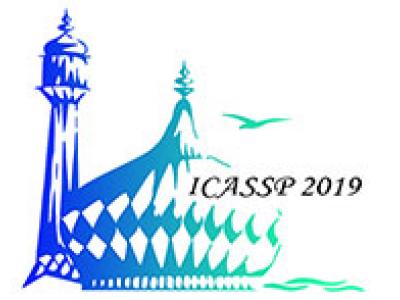
- Citation Author(s):
- Submitted by:
- Jonas Sautter
- Last updated:
- 8 May 2019 - 2:16am
- Document Type:
- Poster
- Document Year:
- 2019
- Event:
- Presenters:
- Jonas Sautter
- Categories:
- Log in to post comments
The aim of artificial bandwidth extension is to recreate wideband speech (0 - 8 kHz) from a narrowband speech signal (0 - 4 kHz). State-of-the-art approaches use neural networks for this task. As a loss function during training, they employ the mean squared error between true and estimated wideband spectra. This, however, comes with the drawback of over-smoothing, which expresses itself in strongly underestimated dynamics of the upper frequency band. We previously proposed to tackle this problem by discriminative training, i.e., a modification of the loss function that is designed to improve the separation between fricatives and vowels. Other authors instead took a generative adversarial network (GAN) approach. This was motivated by the fact that GANs demonstrated big reductions of over-smoothing in speech synthesis. In this work, we combine these two approaches. In particular, we show that conditional GANs improve the speech quality by a CMOS score of 0.28 compared to GANs while the combined approach yields an improvement of 0.84.