Documents
Poster
Attacks On Digital Watermarks For Deep Neural Networks
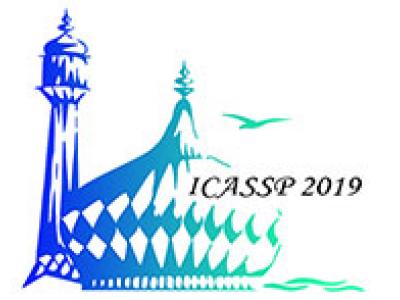
- Citation Author(s):
- Submitted by:
- Tianhao Wang
- Last updated:
- 9 May 2019 - 9:11pm
- Document Type:
- Poster
- Document Year:
- 2019
- Event:
- Presenters:
- Tianhao Wang
- Paper Code:
- 3570
- Categories:
- Log in to post comments
Training deep neural networks is a computationally expensive task. Furthermore, models are often derived from proprietary datasets that have been carefully prepared and labelled. Hence, creators of deep learning models want to protect their models against intellectual property theft. However, this is not always possible, since the model may, e.g., be embedded in a mobile app for fast response times. As a countermeasure watermarks for deep neural networks have been developed that embed secret information into the model. This information can later be retrieved by the creator to prove ownership.
Uchida et al. proposed the first such watermarking method. The advantage of their scheme is that it does not compromise the accuracy of the model prediction. However, in this paper we show that their technique modifies the statistical distribution of the model. Using this modification we can not only detect the presence of a watermark, but even derive its embedding length and use this information to remove the watermark by overwriting it. We show analytically that our detection algorithm follows consequentially from their embedding algorithm and propose a possible countermeasure. Our findings shall help to refine the definition of undetectability of watermarks for deep neural networks.