Documents
Poster
Attentional Road Safety Networks
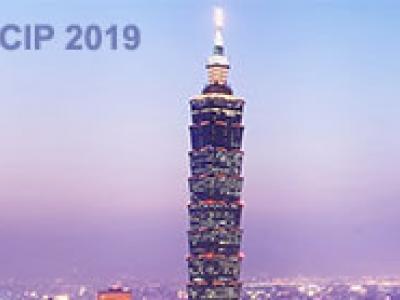
- Citation Author(s):
- Submitted by:
- Deepak Srivatsav
- Last updated:
- 19 September 2019 - 10:38am
- Document Type:
- Poster
- Document Year:
- 2019
- Event:
- Presenters:
- Deepak Srivatsav
- Paper Code:
- MQ.PG.7
- Categories:
- Log in to post comments
Road safety mapping using satellite images is a cost-effective but a challenging problem for smart city planning. The scarcity of labeled data, misalignment and ambiguity makes it hard to learn efficient embeddings in order to classify between safe and dangerous road segments. In this paper, we address the challenges using a region guided attention network. In our model, we extract global features from a base network and augment it with local features obtained using the region guided attention network. In addition, we perform domain adaptation for unlabeled target data. In order to bridge the gap between safe samples and dangerous samples from source and target respectively, we propose a loss function based on within and between class covariance matrices. We conduct experiments on a public dataset of London to show that the algorithm achieves significant results with the classification accuracy of 86.21%. We obtain an increase of 4% accuracy for NYC using domain adaptation network.