Documents
Poster
Attributable Watermarking of Speech Generative Models
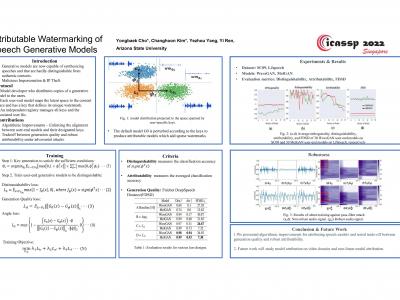
- Citation Author(s):
- Submitted by:
- Yongbaek Cho
- Last updated:
- 16 May 2022 - 11:05pm
- Document Type:
- Poster
- Event:
- Presenters:
- Yongbaek Cho, Chnaghoon Kim, Yezhou Yang, Yi Ren
- Paper Code:
- IFS-8.5
- Categories:
- Log in to post comments
Generative models are now capable of synthesizing images, speeches, and videos that are hardly distinguishable from authentic contents. Such capabilities cause concerns such as malicious impersonation and IP theft. This paper investigates a solution for model attribution, i.e., the classification of synthetic contents by their source models via watermarks embedded in the contents. Building on past success of model attribution in the image domain, we discuss algorithmic improvements for generating user-end speech models that empirically achieve high attribution accuracy, while maintaining high generation quality. We show the tradeoff between attributability and generation quality under a variety of attacks on generated speech signals attempting to remove the watermarks, and the feasibility of learning robust watermarks against these attacks.