Documents
Poster
AUTOMATIC MOTION ARTIFACT DETECTION FOR WHOLE-BODY MAGNETIC RESONANCE IMAGING
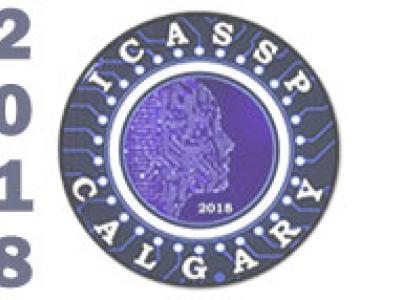
- Citation Author(s):
- Submitted by:
- Thomas Kuestner
- Last updated:
- 12 April 2018 - 12:45pm
- Document Type:
- Poster
- Document Year:
- 2018
- Event:
- Presenters:
- Thomas Kuestner
- Paper Code:
- 1580
- Categories:
- Log in to post comments
Magnetic resonance (MR) plays an important role in medical imaging. It can be flexibly tuned towards different applications for deriving a meaningful diagnosis. However, its long acquisition times and flexible parametrization make it on the other hand prone to artifacts which obscure the underlying image content or can be misinterpreted as anatomy. Patient-induced motion artifacts are still one of the major extrinsic factors which degrade image quality. In this work, an automatic reference-free motion artifact detection, including localization and quantification, is proposed which can be used prospectively as quality control (e.g. scan adjustment) or retrospectively as quality control (e.g. supported diagnosis). The detection is achieved via trained convolutional neural networks (CNN). This study focuses on investigating the optimal CNN architecture and required training set composition to derive a general and robust network for MR motion artifact detection. In a volunteer cohort an average accuracy of 91% was achieved.