Documents
Poster
BAYESIAN-OPTIMIZED BIDIRECTIONAL LSTM REGRESSION MODEL FOR NON-INTRUSIVE LOAD MONITORING
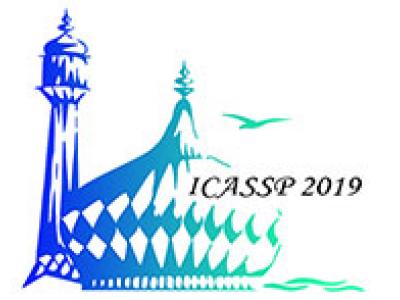
- Citation Author(s):
- Submitted by:
- Maria Kaselimi
- Last updated:
- 10 May 2019 - 4:49pm
- Document Type:
- Poster
- Document Year:
- 2019
- Event:
- Presenters:
- Maria Kaselimi
- Paper Code:
- IDSP-P1.11
- Categories:
- Log in to post comments
In this paper, a Bayesian-optimized bidirectional Long Short -Term Memory (LSTM) method for energy disaggregation, is introduced. Energy disaggregation, or Non-Intrusive Load Monitoring (NILM), is a process aiming to identify the individual contribution of appliances in the aggregate electricity load. The proposed model, Bayes-BiLSTM, is structured in a modular way to address multi-dimensionality issues that arise when the number of appliances increase. In addition, a non-causal model is introduced in order to tackle with inherent structure, characterizing the operation of multi-state appliances. Furthermore, a Bayesian-optimized framework is introduced to select the best configuration of the proposed regression model, thus increasing performance. Experimental results indicate the proposed method’s superiority, compared to the current state-of-the-art.