Documents
Presentation Slides
Benchmarking Adversarial Robustness of Image Shadow Removal with Shadow-adaptive Attacks
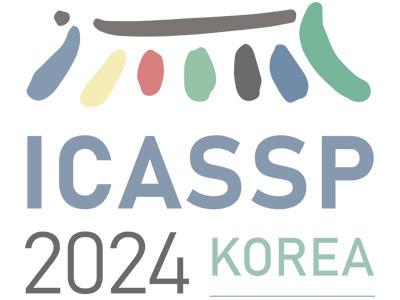
- DOI:
- 10.60864/wt71-4s40
- Citation Author(s):
- Submitted by:
- Chong Wang
- Last updated:
- 6 June 2024 - 10:27am
- Document Type:
- Presentation Slides
- Document Year:
- 2024
- Event:
- Presenters:
- Chong Wang
- Paper Code:
- SS-L5.6
- Categories:
- Log in to post comments
Shadow removal is a task aimed at erasing regional shadows present in images and reinstating visually pleasing natural scenes with consistent illumination. While recent deep learning techniques have demonstrated impressive performance in image shadow removal, their robustness against adversarial attacks remains largely unexplored. Furthermore, many existing attack frameworks typically allocate a uniform budget for perturbations across the entire input image, which may not be suitable for attacking shadow images. This is primarily due to the unique characteristic of spatially varying illumination within shadow images. In this paper, we propose a novel approach, called shadow-adaptive adversarial attack. Different from standard adversarial attacks, our attack budget is adjusted based on the pixel intensity in different regions of shadow images. Consequently, the optimized adversarial noise in the shadowed regions becomes visually less perceptible while permitting a greater tolerance for perturbations in non-shadow regions. The proposed shadow-adaptive attacks naturally align with the varying illumination distribution in shadow images, resulting in perturbations that are less conspicuous. Building on this, we conduct a comprehensive empirical evaluation of existing shadow removal methods, subjecting them to various levels of attack on publicly available datasets.