Documents
Poster
BI-APC: BIDIRECTIONAL AUTOREGRESSIVE PREDICTIVE CODING FOR UNSUPERVISED PRE-TRAINING AND ITS APPLICATION TO CHILDREN’S ASR
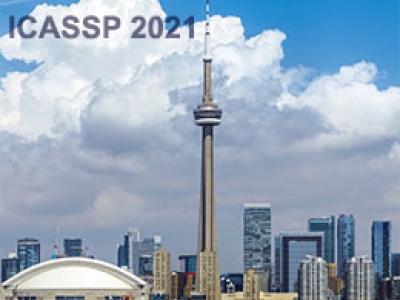
- Citation Author(s):
- Submitted by:
- Ruchao Fan
- Last updated:
- 25 June 2021 - 1:41pm
- Document Type:
- Poster
- Document Year:
- 2021
- Event:
- Presenters:
- Ruchao Fan
- Categories:
- Log in to post comments
We present a bidirectional unsupervised model pre-training (UPT) method and apply it to children’s automatic speech recognition (ASR). An obstacle to improving child ASR is the scarcity of child speech databases. A common approach to alleviate this problem is model pre-training using data from adult speech. Pre-training can be done using supervised (SPT) or unsupervised methods, depending on the availability of annotations. Typically, SPT performs better. In this paper, we focus on UPT to address the situations when pre-training data are unlabeled. Autoregressive predictive coding (APC), a UPT method, predicts frames from only one direction, limiting its use to uni-directional pre-training. Conventional bidirectional UPT methods, however, predict only a small portion of frames. To extend the benefits of APC to bi-directional pre-training, Bi-APC is proposed. We then use adaptation techniques to transfer knowledge learned from adult speech (using the Librispeech corpus) to child speech (OGI Kids corpus). LSTM-based hybrid systems are investigated. For the uni-LSTM structure, APC obtains similar WER improvements to SPT over the baseline. When applied to BLSTM, however, APC is not as competitive as SPT, but our proposed Bi-APC has comparable improvements to SPT.