Documents
Poster
BLENDA: DOMAIN ADAPTIVE OBJECT DETECTION THROUGH DIFFUSION-BASED BLENDING
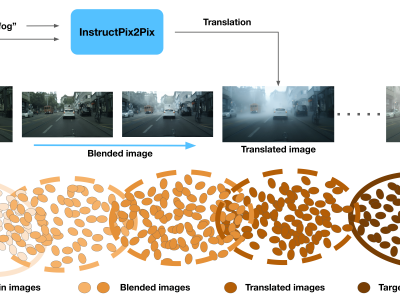
- DOI:
- 10.60864/wftw-8q04
- Citation Author(s):
- Submitted by:
- Tzuhsuan Huang
- Last updated:
- 14 April 2024 - 11:15pm
- Document Type:
- Poster
- Presenters:
- Chen-Che Huang
- Paper Code:
- IVMSP-P17
- Categories:
- Log in to post comments
Unsupervised domain adaptation (UDA) aims to transfer a model learned using labeled data from the source domain to unlabeled data in the target domain. To address the large domain gap issue between the source and target domains, we propose a novel regularization method for domain adaptive object detection, BlenDA, by generating the pseudo samples of the intermediate domains and their corresponding soft domain labels for adaptation training. The intermediate samples are generated by dynamically blending the source images with their corresponding translated images using an off-the-shelf pre-trained text-to-image diffusion model which takes the text label of the target domain as input and has demonstrated superior image-to-image translation quality. Based on experimental results from two adaptation benchmarks, our proposed approach can significantly enhance the performance of the state-of-the-art domain adaptive object detector, Adversarial Query Transformer (AQT). Particularly, in the Cityscapes to Foggy Cityscapes adaptation, we achieve an impressive 53.4% mAP on the Foggy Cityscapes dataset, surpassing the previous state-of-the-art by 1.5%. It is worth noting that our proposed method is also applicable to various paradigms of domain adaptive object detection. The code is available at https://github.com/aiiu-lab/BlenDA