Documents
Presentation Slides
BNMTRANS: A BRAIN NETWORK SEQUENCE-DRIVEN MANIFOLD-BASED TRANSFORMER FOR COGNITIVE IMPAIRMENT DETECTION USING EEG
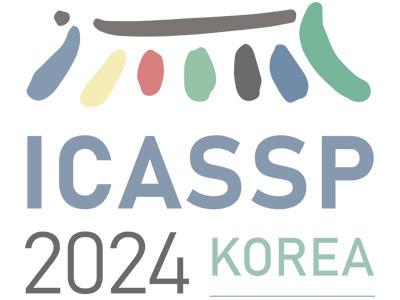
- DOI:
- 10.60864/tsw9-3928
- Citation Author(s):
- Submitted by:
- Z. G. Zhang
- Last updated:
- 6 June 2024 - 10:22am
- Document Type:
- Presentation Slides
- Document Year:
- 2024
- Event:
- Presenters:
- Zhiguo Zhang
- Paper Code:
- BISP-L7.2
- Categories:
- Log in to post comments
Early identification of mild cognitive impairment (MCI) is crucial for the prevention of Alzheimer’s disease. As neurodegenerative
diseases progress, the synchronous activity observed in electroencephalography (EEG) - which indicates functional connectivity -
may change due to the deterioration of the neural system. Thus, developing geometric learning to decode the functional brain structure is essential. Techniques such as graph neural networks and Riemannian manifolds have demonstrated potential in analyzing data with non-Euclidean properties. However, existing methodologies remain insufficient for detecting cognitive impairment effectively. This paper proposes the Brain Network sequence-driven Manifoldbased Transformer (BNMTrans) to identify MCI patterns from EEG data. BNMTrans leverages its strengths by extracting features from sequential brain networks through the self-attention mechanism, guided by the geometric correlations within the Riemannian manifold. Benefiting from the integration of long-term temporal dynamics and structural relationships, this approach outperforms others in EEG feature comparisons and state-of-the-art evaluations based on clinical data from 89 subjects (46 MCI/43 healthy controls) at a local hospital. Our work has significance for both MCI clinical management and technical progression in the EEG field.