Documents
Presentation Slides
Bubblenet: A Disperse Recurrent Structure To Recognize Activities
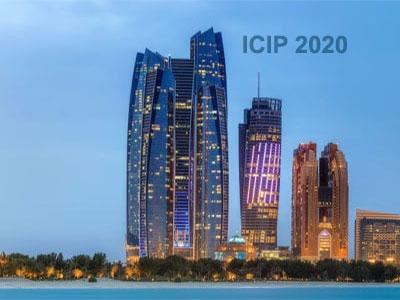
- Citation Author(s):
- Submitted by:
- Igor Bastos
- Last updated:
- 2 November 2020 - 3:16pm
- Document Type:
- Presentation Slides
- Document Year:
- 2020
- Event:
- Presenters:
- Igor L. O. Bastos
- Paper Code:
- 2247
- Categories:
- Log in to post comments
This paper presents an approach to perform human activity recognition in videos through the employment of a deep recurrent network, taking as inputs appearance and optical flow information. Our method proposes a novel architecture named BubbleNET, which is based on a recurrent layer dispersed into several modules (referred to as bubbles) along with an attention mechanism based on squeeze-and-excitation strategy, responsible to modulate each bubble contribution. Thereby, we intend to gather information from fundamentally correlated segments of the input data, creating a signature of components that characterize each activity. Our experiments, conducted on widely employed activity recognition datasets, support the existence of these signatures, evidenced by maps of bubble activations for every class of the datasets. To compare the approach to literature methods, mean accuracy is taken into account, for which BubbleNET obtained 97.62%, 91.70% and 82.60% on UCF-101, YUP++ and HMDB-51 datasets, respectively, being placed among state-of-the-art methods.