Documents
Poster
CAN LLM FIND THE GREEN CIRCLE? INVESTIGATION AND HUMAN-GUIDED TOOL MANIPULATION FOR COMPOSITIONAL GENERALIZATION
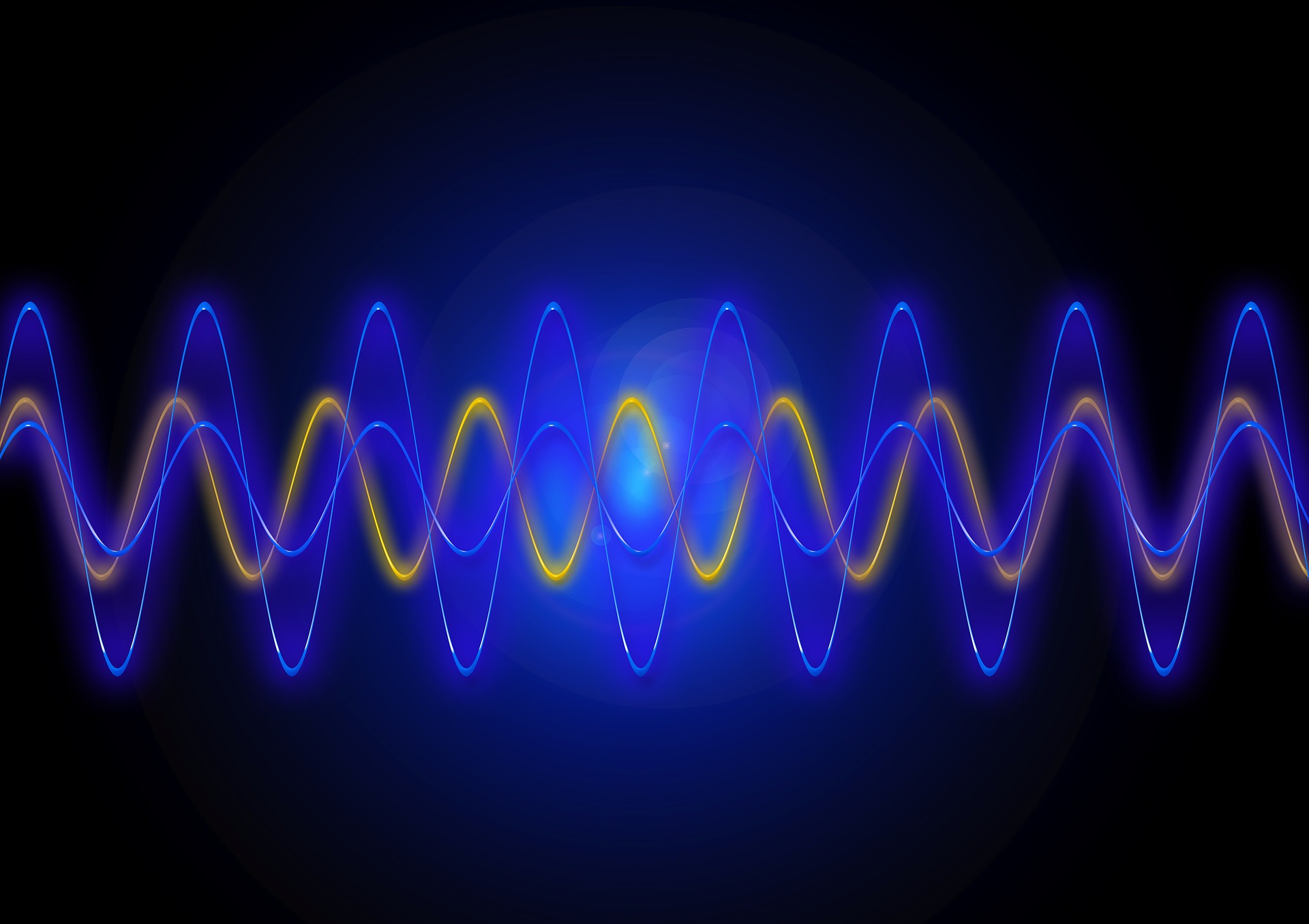
- DOI:
- 10.60864/51gr-pk58
- Citation Author(s):
- Submitted by:
- Min Zhang
- Last updated:
- 6 June 2024 - 10:50am
- Document Type:
- Poster
- Categories:
- Keywords:
- Log in to post comments
The meaning of complex phrases in natural language is composed of their individual components. The task of compositional generalization evaluates a model's ability to understand new combinations of components. Previous studies trained smaller, task-specific models, which exhibited poor generalization. While large language models (LLMs) exhibit impressive generalization abilities on many tasks through in-context learning (ICL), their potential for compositional generalization remains unexplored. In this paper, we first empirically investigate prevailing ICL methods in compositional generalization. We find that they struggle with complex compositional questions due to cumulative errors in long reasoning steps and intricate logic required for tool-making. Consequently, we propose a human-guided tool manipulation framework (HTM) that generates tools for sub-questions and integrates multiple tools. Our method enhances the effectiveness of tool creation and usage with minimal human effort. Experiments show that our method achieves state-of-the-art performance on two compositional generalization benchmarks and outperforms existing methods on the most challenging test split by nearly 70%.