Documents
Poster
CASS-NAT: CTC Alignment-Based Single Step Non-Autoregressive Transformer for Speech Recognition
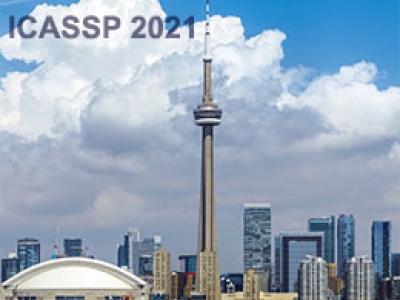
- Citation Author(s):
- Submitted by:
- Ruchao Fan
- Last updated:
- 25 June 2021 - 1:39pm
- Document Type:
- Poster
- Document Year:
- 2021
- Event:
- Presenters:
- Ruchao Fan
- Categories:
- Keywords:
- Log in to post comments
We propose a CTC alignment-based single step non-autoregressive transformer (CASS-NAT) for speech recognition. Specifically, the CTC alignment contains the information of (a) the number of tokens for decoder input, and (b) the time span of acoustics for each token. The information are used to extract acoustic representation for each token in parallel, referred to as token-level acoustic embedding which substitutes the word embedding in autoregressive transformer (AT) to achieve parallel generation in decoder. During inference, an error-based alignment sampling method is proposed to be applied to the CTC output space, reducing the WER and retaining the parallelism as well. Experimental results show that the proposed method achieves WERs of 3.8%/9.1% on Librispeech test clean/other dataset without an external LM, and a CER of 5.8% on Aishell1 Mandarin corpus, respectively 1 . Compared to the AT baseline, the CASS-NAT has a performance reduction on WER, but is 51.2x faster in terms of RTF. When decoding with an oracle CTC alignment, the lower bound of WER without LM reaches 2.3% on the test-clean set, indicating the potential of the proposed method.