Documents
Poster
A CLASSIFICATION-AIDED FRAMEWORK FOR NON-INTRUSIVE SPEECH QUALITY ASSESSMENT
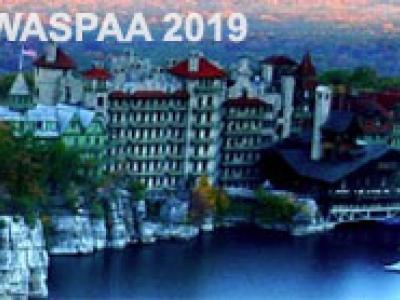
- Citation Author(s):
- Submitted by:
- Donald Williamson
- Last updated:
- 21 October 2019 - 2:28pm
- Document Type:
- Poster
- Document Year:
- 2019
- Event:
- Presenters:
- Donald Williamson
- Paper Code:
- 1570547868
- Categories:
- Keywords:
- Log in to post comments
Objective metrics, such as the perceptual evaluation of speech quality (PESQ) have become standard measures for evaluating speech. These metrics enable efficient and costless evaluations, where ratings are often computed by comparing a degraded speech signal to its underlying clean reference signal. Reference-based metrics, however, cannot be used to evaluate real-world signals that have inaccessible references. This project develops a nonintrusive framework for evaluating the perceptual quality of noisy and enhanced speech. We propose an utterance-level classification-aided non-intrusive (UCAN) assessment approach that combines the task of quality score classification with the regression task of quality score estimation. Our approach uses a categorical quality ranking as an auxiliary constraint to assist with quality score estimation, where we jointly train a multi-layered convolutional neural network in a multi-task manner. This approach is evaluated using the TIMIT speech corpus and several noises under a wide range of signal-to-noise ratios. The results show that the proposed system significantly improves quality score estimation as compared to several state-of-the-art approaches.
WASPAA.v3.pdf
