Documents
Poster
CLOT: Contrastive Learning-Driven and Optimal Transport-Based Training for Simultaneous Clustering
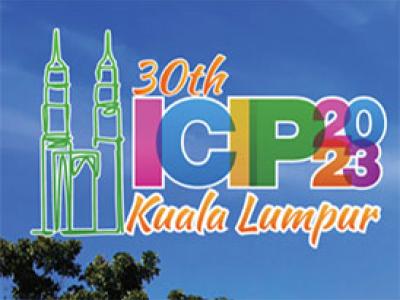
- DOI:
- 10.60864/22wr-5g80
- Citation Author(s):
- Submitted by:
- Mohammed Aburidi
- Last updated:
- 17 November 2023 - 12:05pm
- Document Type:
- Poster
- Document Year:
- 2023
- Event:
- Presenters:
- Roummel Marcia
- Paper Code:
- 2068
- Categories:
- Log in to post comments
Clustering via representation learning is one of the most promising approaches for self-supervised learning of deep neural networks. It aims at obtaining artificial supervisory signals from unlabeled data. In this paper, we propose an online clustering method called CLOT (\underline{C}ontrastive \underline{L}earning-Driven and \underline{O}ptimal \underline{T}ransport-Based Clustering) that is based on robust and multiple losses training settings. More specifically, CLOT learns representations by contrasting both the features at the latent space and the cluster assignments. In the first stage, CLOT performs the instance- and cluster-level contrastive learning which is respectively conducted by maximizing the similarities of the projections of positive pairs (views of the same image) while minimizing those of negative ones (views of the rest of images). In the second stage, it extends standard cross-entropy minimization to an optimal transport problem and solves it using a fast variant of the Sinkhorn-Knopp algorithm to produce the cluster assignments. Further, it enforces consistency between the produced assignments obtained from views of the same image. Compared to the state of the arts, the proposed CLOT outperforms eight competitive clustering methods on three challenging benchmarks, namely, CIFAR-100, STL-10, and ImageNet-10 for ResNet-34.