Documents
Presentation Slides
Coincident Peak Prediction Using a Feed-Forward Neural Network
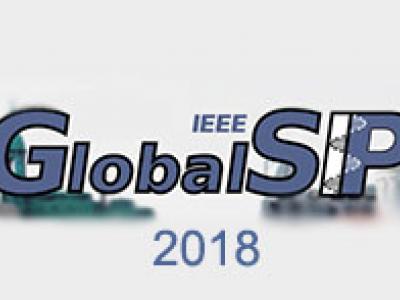
- Citation Author(s):
- Submitted by:
- Chase Dowling
- Last updated:
- 28 November 2018 - 2:08pm
- Document Type:
- Presentation Slides
- Document Year:
- 2018
- Event:
- Presenters:
- Chase Dowling
- Paper Code:
- 1298
- Categories:
- Log in to post comments
A significant portion of a business' annual electrical payments can be made up of coincident peak charges: a transmission surcharge for power consumed when the entire system is at peak demand. This charge occurs only a few times annually, but with per-MW prices orders of magnitudes higher than non-peak times. A business is incentivized to reduce its power consumption, but accurately predicting the timing of peak demand charges is nontrivial. In this paper we present a decision framework based on predicting the day-ahead likelihood of peak demand charges. We train a feed-forward neural network to estimate the probability of system demand peaks and show it outperforms conventional forecasting methods using historical load. Using ERCOT demand and weather data from 2010-2017, we show the effectiveness of our framework.