Documents
Presentation Slides
COMBINING DEEP EMBEDDINGS OF ACOUSTIC AND ARTICULATORY FEATURES FOR SPEAKER IDENTIFICATION
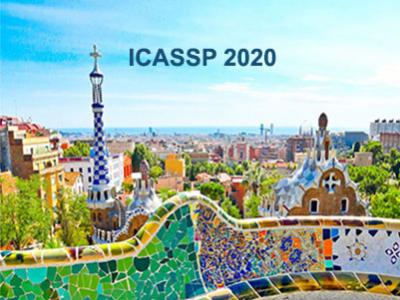
- Citation Author(s):
- Submitted by:
- Chung-Hsien Wu
- Last updated:
- 16 May 2020 - 12:02am
- Document Type:
- Presentation Slides
- Event:
- Categories:
- Log in to post comments
In this study, deep embedding of acoustic and articulatory features are combined for speaker identification. First, a convolutional neural network (CNN)-based universal background model (UBM) is constructed to generate acoustic feature (AC) embedding. In addition, as the articulatory features (AFs) represent some important phonological properties during speech production, a multilayer perceptron (MLP)-based AF embedding extraction model is also constructed for AF embedding extraction. The extracted AC and AF embeddings are concatenated as a combined feature vector for speaker identification using a fully-connected neural network. This proposed system was evaluated by three corpora consisting of King-ASR, LibriSpeech and SITW, and the experiments were conducted according to the properties of the datasets. We adopted all three corpora to evaluate the effect of AF embedding, and the results showed that combining AF embedding into the input feature vector improved the performance of speaker identification. The LibriSpeech corpus was used to evaluate the effect of the number of enrolled speakers. The proposed system achieved an EER of 7.80% outperforming the method based on x-vector with PLDA (8.25%). And we further evaluated the effect of signal mismatch using the SITW corpus. The proposed system achieved an EER of 25.19%, which outperformed the other baseline methods.