Documents
Poster
COMPACT CONVOLUTIONAL RECURRENT NEURAL NETWORKS VIA BINARIZATION FOR SPEECH EMOTION RECOGNITION
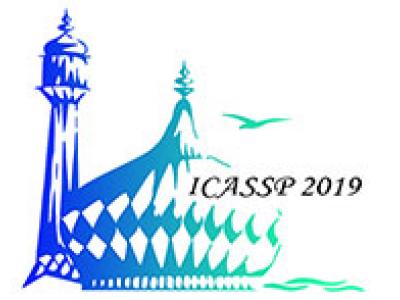
- Citation Author(s):
- Submitted by:
- Yufeng Xiao
- Last updated:
- 7 May 2019 - 7:10pm
- Document Type:
- Poster
- Document Year:
- 2019
- Event:
- Paper Code:
- ICASSP19005
- Categories:
- Keywords:
- Log in to post comments
Despite the great advances, most of the recently developed automatic speech recognition systems focus on working in a server-client manner, and thus often require a high computational cost, such as the storage size and memory accesses. This, however, does not satisfy the increasing demand for a succinct model that can run smoothly in embedded devices like smartphones. To this end, in this paper we propose a neural network compression method, in the way of quantizing the weights of the neural networks from the original full-precised values into binary values that then can be stored and processed with only one bit per value. In doing this, the traditional neural network-based large-size speech emotion recognition models can be greatly compressed into smaller ones, which demand lower computational cost. To evaluate the feasibility of the proposed approach, we take a state-of-the-art speech emotion recognition model, \ie convolutional recurrent neural networks, as an example, and conduct experiments on two widely used emotional databases. We find that the proposed binary neural networks are able to yield a remarkable model compression rate but at limited expense of model performance.