Documents
Presentation Slides
Conditional Mutual Information Neural Estimator
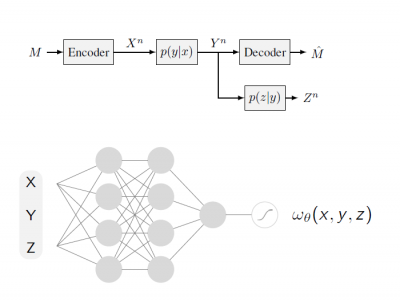
- Citation Author(s):
- Submitted by:
- Sina Molavipour
- Last updated:
- 14 May 2020 - 4:46am
- Document Type:
- Presentation Slides
- Document Year:
- 2020
- Event:
- Presenters:
- Sina Molavipour
- Categories:
- Log in to post comments
Several recent works in communication systems have proposed to leverage the power of neural networks in the design of encoders and decoders. In this approach, these blocks can be tailored to maximize the transmission rate based on aggregated samples from the channel. Motivated by the fact that, in many communication schemes, the achievable transmission rate is determined by a conditional mutual information term, this paper focuses on neural-based estimators for this information-theoretic quantity. Our results are based on variational bounds for the KL-divergence and, in contrast to some previous works, we provide a mathematically rigorous lower bound. However, additional challenges with respect to the unconditional mutual information emerge due to the presence of a conditional density function which we address here.