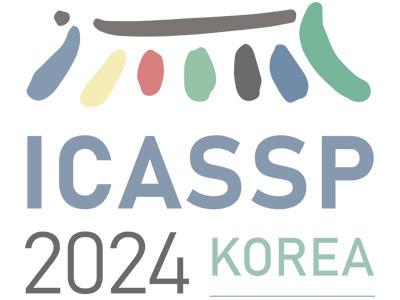
- Read more about Slides for Renyi Divergences Learning for explainable classification of SAR Image Pairs
- Log in to post comments
We consider the problem of classifying a pair of Synthetic Aperture Radar (SAR) images by proposing an explainable and frugal algorithm that integrates a set of divergences. The approach relies on a statistical framework that takes standard probability distributions into account for modelling SAR data. Then, by learning a combination of parameterized Renyi divergences and their parameters from the data, we are able to classify the pair of images with fewer parameters than regular machine learning approaches while also allowing an interpretation of the results related to the priors used.
- Categories:
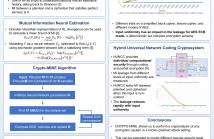
- Read more about CRYPTO-MINE: Cryptanalysis via Mutual Information Neural Estimation
- Log in to post comments
The use of Mutual Information (MI) as a measure to evaluate the efficiency of cryptosystems has an extensive history. However, estimating MI between unknown random variables in a high-dimensional space is challenging. Recent advances in machine learning have enabled progress in estimating MI using neural networks. This work presents a novel application of MI estimation in the field of cryptography. We propose applying this methodology directly to estimate the MI between plaintext and ciphertext in a chosen plaintext attack.
CryptoMine.pdf

- Categories:
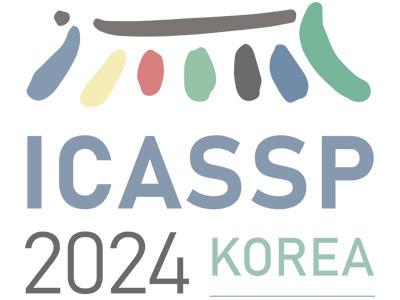
- Read more about Stage-Regularized Neural Stein Critics for Testing Goodness-of-Fit of Generative Models
- Log in to post comments
Learning to differentiate model distributions from observed data is a fundamental problem in statistics and machine learning, and high-dimensional data remains a challenging setting for such problems. Metrics that quantify the disparity in probability distributions, such as the Stein discrepancy, play an important role in high-dimensional statistical testing. This paper presents a method based on neural network Stein critics to distinguish between data sampled from an unknown probability distribution and a nominal model distribution with a novel staging of the weight of regularization.
- Categories:
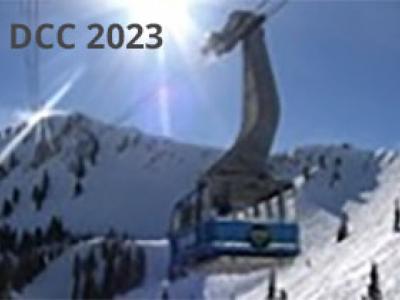
- Read more about Rate-Distortion via Energy-Based Models
- Log in to post comments
- Categories:
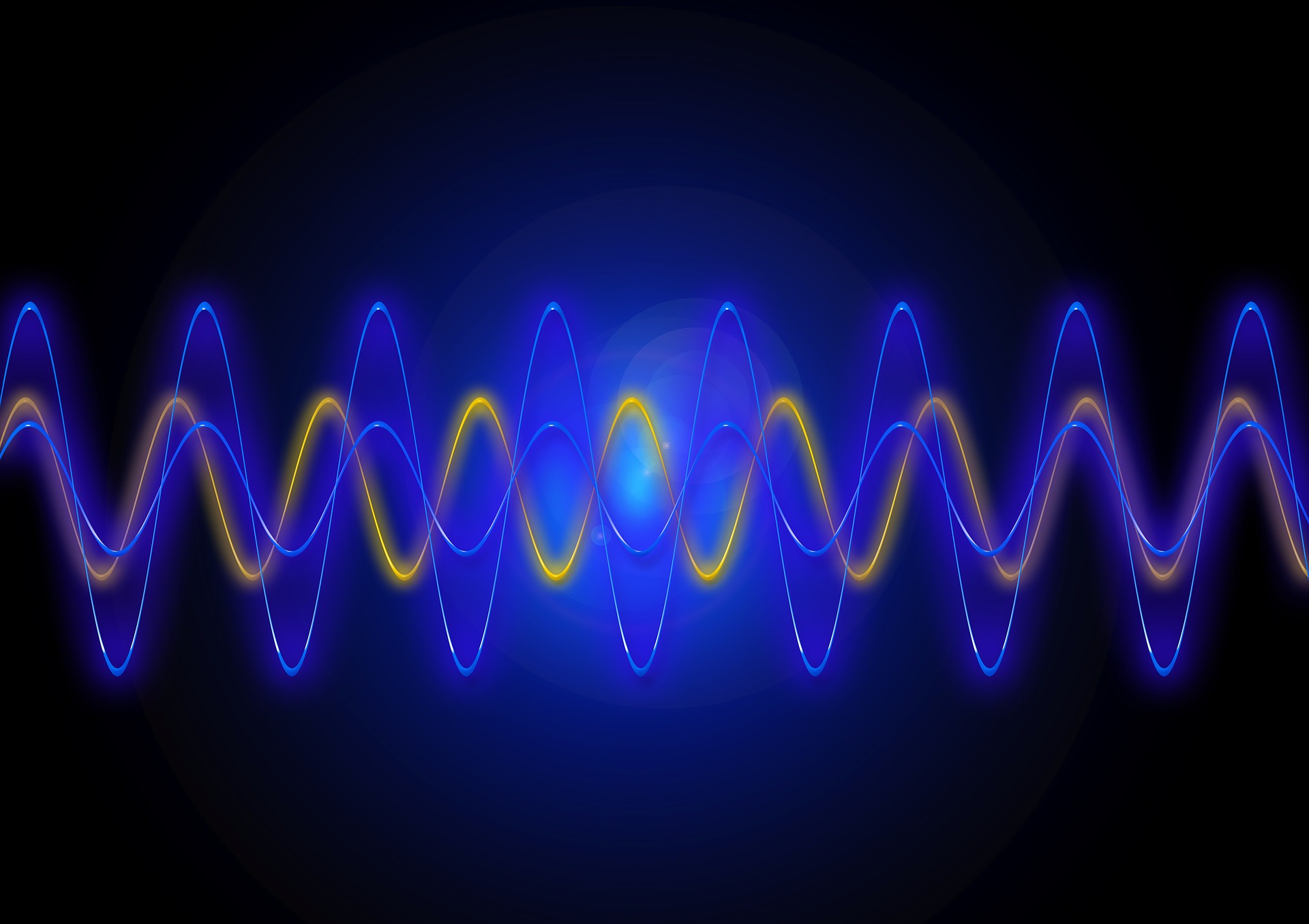
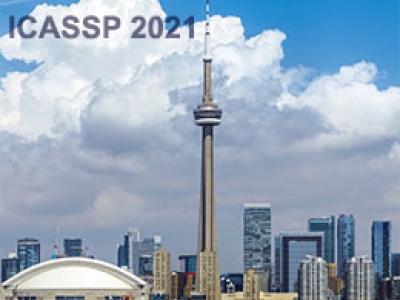
- Read more about Deep Deterministic Information Bottleneck with Matrix-Based Entropy Functional
- Log in to post comments
We introduce the matrix-based Renyi’s α-order entropy functional to parameterize Tishby et al. information bottleneck (IB) principle with a neural network. We term our methodology Deep Deterministic Information Bottleneck (DIB), as it avoids variational inference and distribution assumption. We show that deep neural networks trained with DIB outperform the variational objective counterpart and those that are trained
with other forms of regularization, in terms of generalization performance and robustness to adversarial attack. Code available at
- Categories:
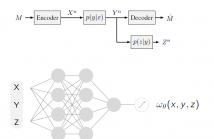
- Read more about Conditional Mutual Information Neural Estimator
- Log in to post comments
Several recent works in communication systems have proposed to leverage the power of neural networks in the design of encoders and decoders. In this approach, these blocks can be tailored to maximize the transmission rate based on aggregated samples from the channel. Motivated by the fact that, in many communication schemes, the achievable transmission rate is determined by a conditional mutual information term, this paper focuses on neural-based estimators for this information-theoretic quantity.
- Categories:
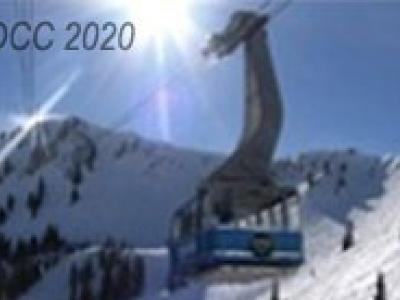
- Read more about Denoising Deep Boltzmann Machines: Compression for Deep Learning
- Log in to post comments
- Categories:
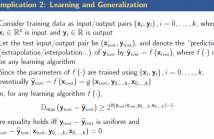
- Read more about Generic Bounds on the Maximum Deviations in Sequential/Sequence Prediction (and the Implications in Recursive Algorithms and Learning/Generalization)
- Log in to post comments
In this paper, we derive generic bounds on the maximum deviations in prediction errors for sequential prediction via an information-theoretic approach. The fundamental bounds are shown to depend only on the conditional entropy of the data point to be predicted given the previous data points. In the asymptotic case, the bounds are achieved if and only if the prediction error is white and uniformly distributed.
- Categories:
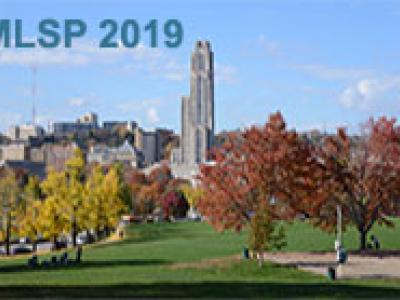
- Read more about Minimax Active Learning via Minimal Model Capacity
- Log in to post comments
Active learning is a form of machine learning which combines supervised learning and feedback to minimize the training set size, subject to low generalization errors. Since direct optimization of the generalization error is difficult, many heuristics have been developed which lack a firm theoretical foundation. In this paper, a new information theoretic criterion is proposed based on a minimax log-loss regret formulation of the active learning problem. In the first part of this paper, a Redundancy Capacity theorem for active learning is derived along with an optimal learner.
- Categories: