Documents
Poster
CONTINUOUS HAND GESTURE SPOTTING AND CLASSIFICATION USING 3D FINGER JOINTS INFORMATION
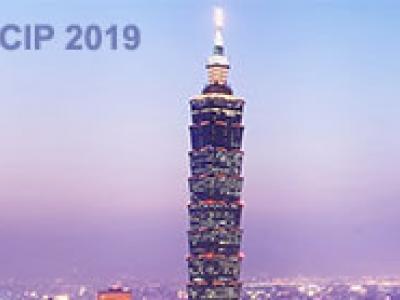
- Citation Author(s):
- Submitted by:
- gueesang lee
- Last updated:
- 17 September 2019 - 2:55am
- Document Type:
- Poster
- Document Year:
- 2019
- Event:
- Presenters:
- GUEESANG LEE
- Paper Code:
- ICIP 2019 Paper #1745
- Categories:
- Log in to post comments
This paper presents a novel approach for continuous dynamic hand gesture recognition for RGB video input. Our approach contains two main modules. Firstly, in the gesture spotting module, the video sequence with continuous gestures are pre-segmented into isolated gestures. Secondly, the gesture classification module classifies the segmented gestures. In the gesture spotting module, the motion of the hand palm and finger movements are fed into Bidirectional Long Short-Term Memory (Bi-LSTM) network for gesture spotting purpose. In the gesture classification module, two 3D Convolution Neural Networks (3D_CNN) and one Long Short-Term Memory (LSTM) network are combined to efficiently utilize the combination of multiple data channels such as RGB, Optical Flow and 3D key pose positions. The promising performance of our approach is obtained by experiments conducted on three publish datasets – Chalearn LAP ConGD dataset, 20BN-Jester, and NVIDIA Dynamic Hand gesture Dataset. Our approach achieves mean Jaccard Index of 0.5535, which outperforms the state-of-the-art methods on Chalearn LAP ConGD challenge.