Documents
Poster
Convex Neural Autoregressive Models: Towards Tractable, Expressive, and Theoretically-Backed Models for Sequential Forecasting and Prediction
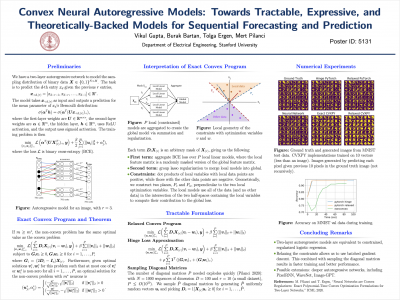
- Citation Author(s):
- Submitted by:
- Vikul Gupta
- Last updated:
- 23 September 2021 - 11:56pm
- Document Type:
- Poster
- Document Year:
- 2021
- Event:
- Presenters:
- Vikul Gupta
- Paper Code:
- 5131
- Categories:
- Log in to post comments
Three features are crucial for sequential forecasting and generation models: tractability, expressiveness, and theoretical backing. While neural autoregressive models are relatively tractable and offer powerful predictive and generative capabilities, they often have complex optimization landscapes, and their theoretical properties are not well understood. To address these issues, we present convex formulations of autoregressive models with one hidden layer. Specifically, we prove an exact equivalence between these models and constrained, regularized logistic regression by using semi-infinite duality to embed the data matrix onto a higher dimensional space and introducing inequality constraints. To make this formulation tractable, we approximate the constraints using a hinge loss or drop them altogether. Furthermore, we demonstrate faster training and competitive performance of these implementations compared to their neural network counterparts on a variety of data sets. Consequently, we introduce techniques to derive tractable, expressive, and theoretically-interpretable models that are nearly equivalent to neural autoregressive models.