Documents
Poster
CROSS-LAYER AGGREGATION WITH TRANSFORMERS FOR MULTI-LABEL IMAGE CLASSIFICATION
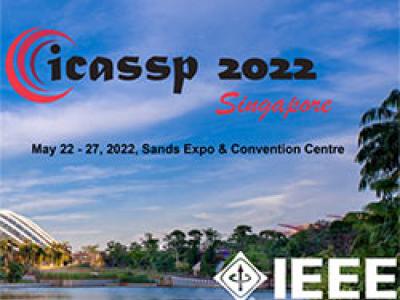
- Citation Author(s):
- Submitted by:
- Weibo Zhang
- Last updated:
- 8 May 2022 - 11:17pm
- Document Type:
- Poster
- Document Year:
- 2022
- Event:
- Presenters:
- Weibo Zhang
- Paper Code:
- MLSP-12.6
- Categories:
- Log in to post comments
Multi-label image classification task aims to predict multiple object labels in a given image and faces the challenge of variable-sized objects. Limited by the size of CNN convolution kernels, existing CNN-based methods have difficulty
capturing global dependencies and effectively fusing multiple layers features, which is critical for this task. Recently, transformers have utilized multi-head attention to extract feature with long range dependencies. Inspired by this, this
paper proposes a Cross-layer Aggregation with Transformers (CAT) framework, which leverages transformers to capture the long range dependencies of CNN-based features with Long Range Dependencies module and aggregate the features layer by layer with Cross-Layer Fusion module. To make the framework efficient, a multi-head pre-max attention is designed to reduce the computation cost when fusing the high-resolution features of lower-layers. On two widely-used benchmarks (i.e., VOC2007 and MS-COCO), CAT provides a stable improvement over the baseline and produces a competitive performance.