Documents
Poster
CrowNN: Human-in-the-loop Network with Crowd-generated Inputs
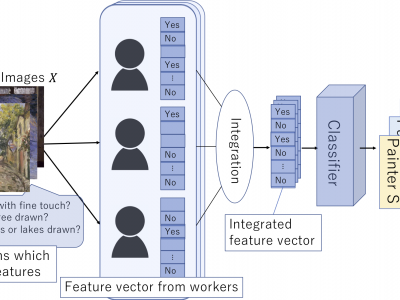
- Citation Author(s):
- Submitted by:
- Yusuke Sakata
- Last updated:
- 10 May 2019 - 4:36am
- Document Type:
- Poster
- Document Year:
- 2019
- Event:
- Presenters:
- Yusuke Sakata
- Paper Code:
- BD-P2
- Log in to post comments
Input features are indispensable for almost all machine learning methods; however, their definitions themselves are sometimes too abstract to extract automatically. Human-in-the- loop machine learning is a promising solution to such cases where humans extract the feature values for machine learning models. We use crowdsourcing for feature value extraction and consider a problem to aggregate the feature values to improve machine learning classifiers. We propose a novel neural network model called CROWNN, a neural network with crowd-generated inputs with the worker convolution layer, that learns both the capabilities of human feature extractors and the weights of a neural network classifier by applying the idea of the convolution neural network to feature aggregation. Our experiments using four datasets show the proposed method outperforms the baseline method using unsupervised aggregation methods in some datasets. We also show the robustness of the proposed model against the existence of spam workers, especially when they are malicious workers who intentionally flip the feature values.