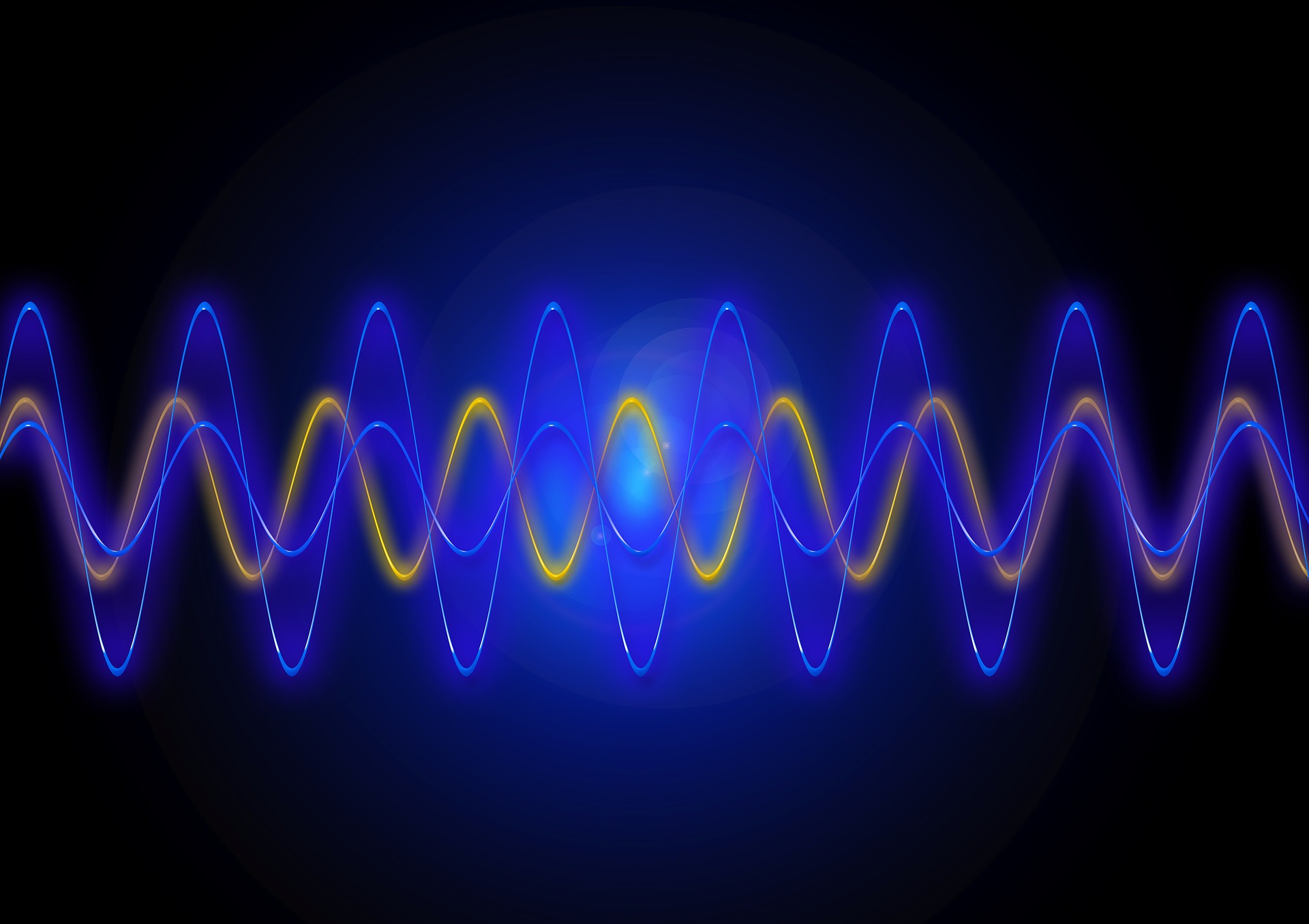
- Read more about BRIDGING THE GAP: A SELF-LEARNING MODEL USING IMPLICIT KNOWLEDGE FOR CHINESE SPELLING CORRECTION
- Log in to post comments
Chinese Spelling Correction (CSC) is a challenging and essential task in natural language processing. In this study, we introduces a new method for Chinese Spelling Correction (CSC) that addresses three unattended areas in prior studies. Firstly, we use an Implicit Knowledge Extraction Network to overcome limitations of conventional methods that rely on explicit knowledge alone. Secondly, we use KL divergence to limit the effect of incorrect characters on semantic understanding, ensuring consistent meaning.
- Categories:
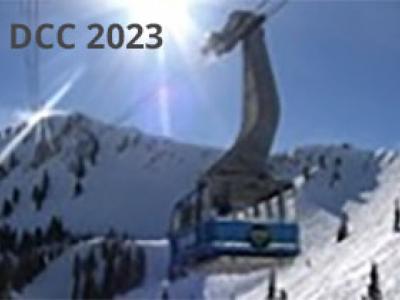
- Categories:
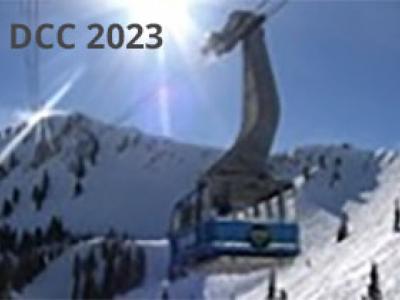
- Read more about Model Compression for Data Compression: Neural Network Based Lossless Compressor Made Practical
- Log in to post comments
- Categories:
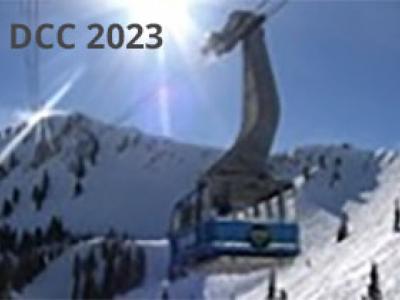
Recently, Quantum Annealing (QA) has attracted attention as an efficient algorithm for combinatorial optimization problems. In QA, the input data size becomes large and its reduction is important for accelerating by the hardware emulation since the usable memory size and its bandwidth are limited. The paper proposes the compression method of input sparse matrices for QA emulator. The proposed method uses the sparseness of the coefficient matrix and the reappearance of the same values.
- Categories:
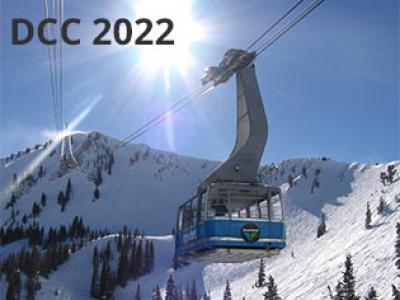
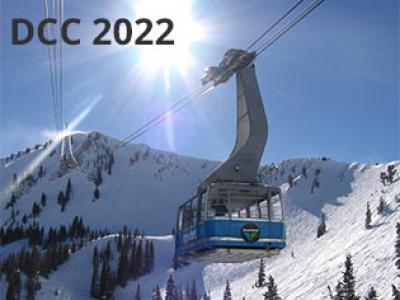
- Read more about Applying Practical Parallel Grammar Compression to Large-scale Data
- Log in to post comments
Re-pair is a grammar-based compression algorithm. It achieves higher compression rates for text, graph, and tree. While Re-pair is a linear-time algorithm, it is slower than other general compression algorithms in practice. This is an obstacle in applying Re-pair to large-scale data. In this paper, we present Parallel Re-pair, a practical implementation that enables parallel processing of Re-pair. In Parallel Re-pair, Re-pair is executed in multiple threads for the divided block. Each thread shares a dictionary and it can output a single CFG.
- Categories:
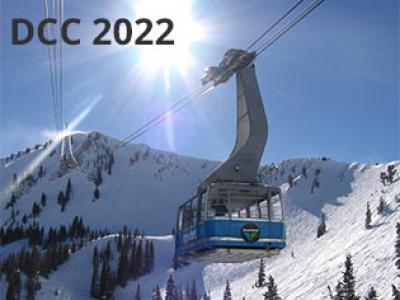
- Read more about Chunk Content is not Enough: Chunk-Context Aware Resemblance Detection for Deduplication Delta Compression
- Log in to post comments
With the growing popularity of cloud storage, identifying and removing duplicate data across users is getting more critical for service providers. Thus, many researchers have attracted attention for data resemblance to detect redundancy among similar data. It uses feature extraction to detect data chunks with high similarity first, and then treat them as candidates for removing redundancy.
- Categories:
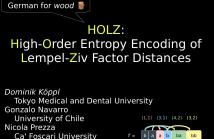
- Read more about HOLZ: High-Order Entropy Encoding of Lempel-Ziv Factor Distances
- Log in to post comments
We propose a new representation of the offsets of the Lempel-Ziv (LZ) factorization
based on the co-lexicographic order of the text's prefixes.
The selected offsets tend to approach the k-th order empirical entropy.
Our evaluations show that this choice is superior to
the rightmost and bit-optimal LZ parsings on datasets with small high-order entropy.
- Categories:
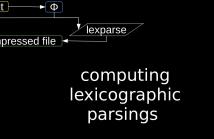
- Read more about Computing Lexicographic Parsings
- 2 comments
- Log in to post comments
We give memory-friendly algorithms computing the compression schemes lexparse in linear time.
- Categories:
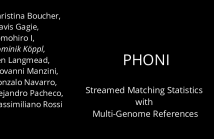
- Read more about PHONI: Streamed Matching Statistics with Multi-Genome References
- Log in to post comments
Computing the matching statistics of patterns with respect to a text is a fundamental task in bioinformatics, but a formidable one when the text is a highly compressed genomic database. Bannai et al. gave an efficient solution for this case, which Rossi et al. recently implemented, but it uses two passes over the patterns and buffers a pointer for each character during the first pass. In this paper, we simplify their solution and make it streaming, at the cost of slowing it down slightly.
dcc21phoni.s.pdf

- Categories: