Documents
Presentation Slides
Custom attribution loss for improving generalization and interpretability of deepfake detection
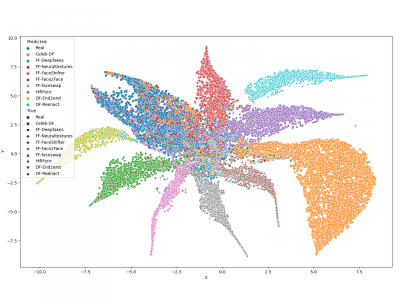
- Citation Author(s):
- Submitted by:
- Pavel Korshunov
- Last updated:
- 6 May 2022 - 9:10am
- Document Type:
- Presentation Slides
- Document Year:
- 2022
- Event:
- Presenters:
- Pavel Korshunov
- Paper Code:
- SS-13.6
- Categories:
- Log in to post comments
The simplicity and accessibility of tools for generating deepfakes pose a significant technical challenge for their detection and filtering. Many of the recently proposed methods for deeptake detection focus on a `blackbox' approach and therefore suffer from the lack of any additional information about the nature of fake videos beyond the fake or not fake labels. In this paper, we approach deepfake detection by solving the related problem of attribution, where the goal is to distinguish each separate type of a deepfake attack. We design a training approach with customized Triplet and ArcFace losses that allow to improve the accuracy of deepfake detection on several publicly available datasets, including Google and Jigsaw, FaceForensics++, HifiFace, DeeperForensics, Celeb-DF, DeepfakeTIMIT, and DF-Mobio. Using an example of Xception net as an underlying architecture, we also demonstrate that when trained for attribution, the model can be used as a tool to analyze the deepfake space and to compare it with the space of original videos.