Documents
Poster
CUTENSOR-TUBAL: OPTIMIZED GPU LIBRARY FOR LOW-TUBAL-RANK TENSORS
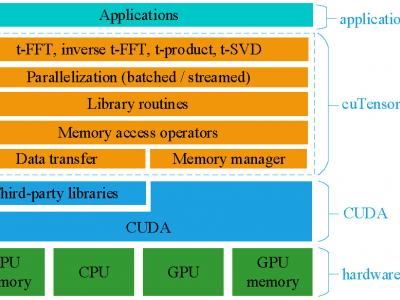
- Citation Author(s):
- Submitted by:
- Tao Zhang
- Last updated:
- 8 May 2019 - 8:21am
- Document Type:
- Poster
- Document Year:
- 2019
- Event:
- Presenters:
- HAI LI
- Paper Code:
- 2071
- Categories:
- Log in to post comments
In this paper, we optimize the computations of third-order low-tubal-rank tensor operations on many-core GPUs. Tensor operations are compute-intensive and existing studies optimize such operations in a case-by-case manner, which can be inefficient and error-prone. We develop and optimize a BLAS-like library for the low-tubal-rank tensor model called cuTensor-tubal, which includes efficient GPU primitives for tensor operations and key processes. We compute tensor operations in the frequency domain and fully exploit tube-wise and slice-wise parallelisms. We design, implement, and optimize four key tensor operations namely t-FFT, inverse t-FFT, t-product, and t-SVD. For t-product and t-SVD, cuTensor-tubal demonstrates significant speedups: maximum 29.16X, 6.72X speedups over the non-optimized GPU counterparts, and maximum 16.91X and 27.03X speedups over the CPU implementations running on dual 10-core Xeon CPUs.
Paper link on IEEE Xplore:https://ieeexplore.ieee.org/document/8682323