Documents
Poster
Cycle-consistent adversarial networks for non-parallel vocal effort based speaking style conversion
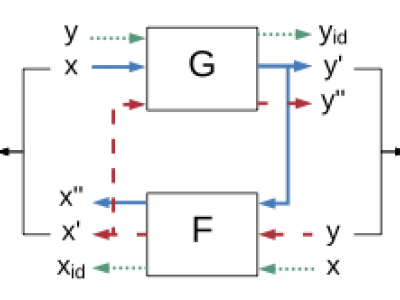
- Citation Author(s):
- Submitted by:
- Shreyas Seshadri
- Last updated:
- 8 May 2019 - 4:29am
- Document Type:
- Poster
- Document Year:
- 2019
- Event:
- Presenters:
- Shreyas Seshadri
- Paper Code:
- 2994
- Categories:
- Log in to post comments
Speaking style conversion (SSC) is the technology of converting natural speech signals from one style to another. In this study, we propose the use of cycle-consistent adversarial networks (CycleGANs) for converting styles with varying vocal effort, and focus on conversion between normal and Lombard styles as a case study of this problem. We propose a parametric approach that uses the Pulse Model in Log domain (PML) vocoder to extract speech features. These features are mapped using the CycleGAN from utterances in the source style to the corresponding features of target speech. Finally, the mapped features are converted to a Lombard speech waveform with the PML. The CycleGAN was compared in subjective listening tests with 2 other standard mapping methods used in conversion, and the CycleGAN was found to have the best performance in terms of speech quality and in terms of the magnitude of the perceptual change between the two styles.