Documents
Presentation Slides
DATA INCUBATION — SYNTHESIZING MISSING DATA FOR HANDWRITING RECOGNITION
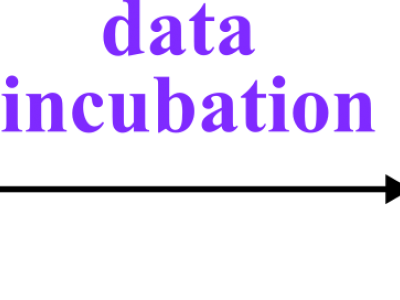
- Citation Author(s):
- Submitted by:
- Jen-Hao Rick Chang
- Last updated:
- 4 May 2022 - 5:35pm
- Document Type:
- Presentation Slides
- Event:
- Presenters:
- Jen-Hao Rick Chang
- Paper Code:
- 3765
- Categories:
- Log in to post comments
In this paper, we demonstrate how a generative model can be used to build a better recognizer through the control of content and style. We are building an online handwriting recognizer from a modest amount of training samples. By training our controllable handwriting synthesizer on the same data, we can synthesize handwriting with previously underrepresented content (e.g., URLs and email addresses) and style (e.g., cursive and slanted). Moreover, we propose a framework to analyze a recognizer that is trained with a mixture of real and synthetic training data. We use the framework to optimize data synthesis and demonstrate significant improvement on handwriting recognition over a model trained on real data only. Overall, we achieve a 66% reduction in Character Error Rate.