Documents
Presentation Slides
Deep Blind Image Quality Assessment by Learning Sensitivity Map
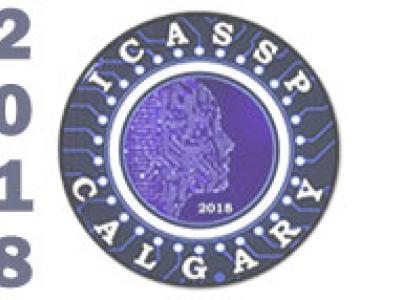
- Citation Author(s):
- Submitted by:
- Sanghoon Lee
- Last updated:
- 20 April 2018 - 1:12am
- Document Type:
- Presentation Slides
- Document Year:
- 2018
- Event:
- Presenters:
- Sanghoon Lee
- Paper Code:
- 1426
- Categories:
- Log in to post comments
Applying a deep convolutional neural network CNN to no reference image quality assessment (NR-IQA) is a challenging task due to the lack of a training database. In this paper, we propose a CNN-based NR-IQA framework that can effectively solve this problem. The proposed method–the Deep Blind image Quality Assessment predictor (DeepBQA)– adopts two-step training stages to avoid overfitting. In the first stage, a ground-truth objective error map is generated and used as a proxy training target. Then, in the second stage, the subjective score is predicted by learning a sensitivity map, which weights each pixel in the predicted objective error map. To compensate the inaccurate prediction of the objective error on the homogeneous regions, we additionally suggest a reliability map. Experiments showed that DeepBQA yields a state-of-the-art correlation with human opinions.