Documents
Presentation Slides
Deep Discovery of Facial Motions using a Shallow Embedding Layer
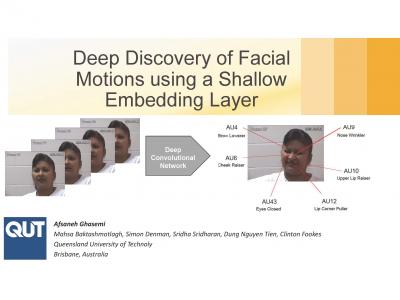
- Citation Author(s):
- Submitted by:
- afsaneh ghasemi...
- Last updated:
- 17 September 2017 - 4:03am
- Document Type:
- Presentation Slides
- Document Year:
- 2017
- Event:
- Presenters:
- Afsaneh Ghasemi
- Paper Code:
- 2171
- Categories:
- Log in to post comments
Unique encoding of the dynamics of facial actions has potential to provide a spontaneous facial expression recognition system. The most promising existing approaches rely on deep learning of facial actions. However, current approaches are often computationally intensive and require a great deal of memory/processing time, and typically the temporal aspect of facial actions are often ignored, despite the potential wealth of information available from the spatial dynamic movements and their temporal evolution over time from neutral state to apex state. To tackle aforementioned challenges, we propose a deep learning framework by using the 3D convolutional filters to extract spatio-temporal features, followed by the LSTM network which is able to integrate the dynamic evolution of short-duration of spatio-temporal features as an emotion progresses from the neutral state to the apex state.
In order to reduce the redundancy of parameters and accelerate the learning of the recurrent neural network, we propose a shallow embedding layer to reduce the number of parameters in the LSTM by up to 98% without sacrificing recognition accuracy. As the fully connected layer approximately contains 95% of the parameters in the network, we decrease the number of parameters in this layer before passing features to the LSTM network, which significantly improves training speed and enables the possibility of deploying a state of the art deep network on real-time applications. We evaluate our proposed
framework on the DISFA and UNBC-McMaster Shoulder pain datasets.