Documents
Poster
DEEP EMBEDDINGS FOR RARE AUDIO EVENT DETECTION WITH IMBALANCED DATA
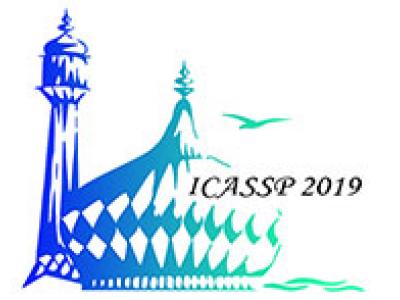
- Citation Author(s):
- Submitted by:
- Vipul Arora
- Last updated:
- 9 July 2019 - 7:29am
- Document Type:
- Poster
- Document Year:
- 2019
- Event:
- Presenters:
- Vipul Arora
- Paper Code:
- MLSP-P7.3
- Categories:
- Log in to post comments
In this paper, we present a method to handle data imbalance for classification with neural networks, and apply it to acoustic event detection (AED) problem. The common approach to tackle data imbalance is to use class-weights in the objective function while training. An existing more sophisticated approach is to map the input to clusters in an embedding space, so that learning is locally balanced by incorporating inter-cluster and inter-class margins. On these lines, we propose a method to learn the embedding using a novel objective function, called triple-header cross entropy. Our scheme integrates in a simple way with back-propagation based training, and is computationally more efficient than general hinge-loss based embedding learning schemes. The empirical evaluation results demonstrate the effectiveness of the proposed method for AED with imbalanced training data.