Documents
Poster
Poster
Deep learning for Minimal Context Classification of Block-types through Side-Channel Analysis
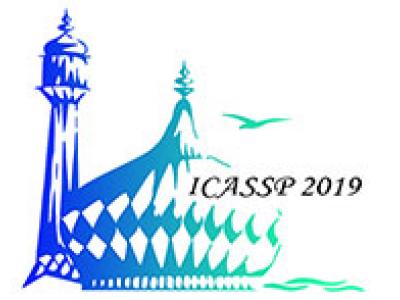
- Citation Author(s):
- Submitted by:
- Louis Jensen
- Last updated:
- 9 May 2019 - 8:19am
- Document Type:
- Poster
- Document Year:
- 2019
- Event:
- Presenters:
- Louis Jensen
- Paper Code:
- 3484
- Categories:
- Log in to post comments
It is well known that electromagnetic and power side-channel attacks allow extraction of unintended information from a computer processor. However, little work has been done to quantify how small a sample is needed in order to glean meaningful information about a program's execution. This paper quantifies this minimum context by training a deep-learning model to track and classify program block types given small windows of side-channel data. We show that a window containing approximately four clock cycles suffices to predict block type with our experimental setup. This implies a high degree of information leakage through side channels, allowing for the external monitoring of embedded systems and Internet of Things devices.