Documents
Presentation Slides
Deep Residual Learning for Model-Based Iterative CT Reconstruction using Plug-and-Play Framework
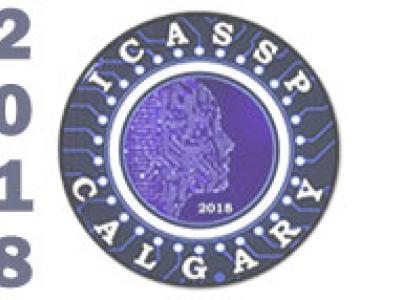
- Citation Author(s):
- Submitted by:
- Dong Hye Ye
- Last updated:
- 19 April 2018 - 7:12pm
- Document Type:
- Presentation Slides
- Document Year:
- 2018
- Event:
- Presenters:
- Dong Hye Ye
- Paper Code:
- 1370
- Categories:
- Keywords:
- Log in to post comments
Model-Based Iterative Reconstruction (MBIR) has shown promising results in clinical studies as they allow significant
dose reduction during CT scans while maintaining the diagnostic image quality. MBIR improves the image quality over analytical reconstruction by modeling both the sensor (e.g., forward model) and the image being reconstructed (e.g., prior model). While the forward model is typically based on the physics of the sensor, accurate prior modeling remains a challenging problem. Markov Random Field (MRF) has been widely used as prior models in MBIR due to simple structure, but they cannot completely capture the subtle characteristics of complex images. To tackle this challenge, we generate a prior model by learning the desirable image property from a large dataset. Toward this, we use Plug-and-Play (PnP) framework which decouples the forward model and the prior model in the optimization procedure, replacing the prior model optimization by a image denoising operator. Then, we adopt the state-of-the-art deep residual learning for the image denoising operator which represents the prior model
in MBIR. Experimental results on real CT scans demonstrate that our PnP MBIR with deep residual learning prior significantly
reduces the noise and artifacts compared to analytical reconstruction and standard MBIR with MRF prior.