Documents
Presentation Slides
DENOISING-ORIENTED DEEP HIERARCHICAL REINFORCEMENT LEARNING FOR NEXT-BASKET RECOMMENDATION
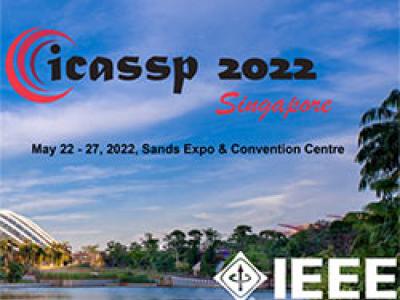
- Citation Author(s):
- Submitted by:
- qihan Du
- Last updated:
- 10 May 2022 - 1:36am
- Document Type:
- Presentation Slides
- Document Year:
- 2022
- Event:
- Presenters:
- Qihan Du
- Paper Code:
- MLSP-35.1
- Categories:
- Keywords:
- Log in to post comments
Next basket recommendation aims to provide users a basket of items on the next visit by considering the sequence of their historical baskets. However, since a user's purchase interests vary over time, historical baskets often contain many irrelevant items to his/her next choices. Therefore, it is necessary to denoise the sequence of historical baskets and reserve the indeed relevant items to enhance the recommendation performance. In this work, we propose a Hierarchical Reinforcement Learning framework for next Basket recommendation, named HRL4Ba, which learns the personalized inter-basket and intra-basket contexts of the user for dynamic denoising. Specifically, the high-level and the low-level agent in the denoising module perform hierarchical decisions, i.e., revise baskets and remove items; the recommendation module serves as the environment to give feedback to agents and recommends the next basket. Extensive experiments on two e-commerce datasets show the HRL4Ba outperforms existing state-of-the-art methods, and our ablation studies further show the effectiveness of each component in HRL4Ba.
HRL4Ba.pptx
