Documents
Poster
DEPTH HUMAN ACTION RECOGNITION BASED ON CONVOLUTION NUERAL NETWORKS AND PRINCIPAL COMPONENT ANALYSIS
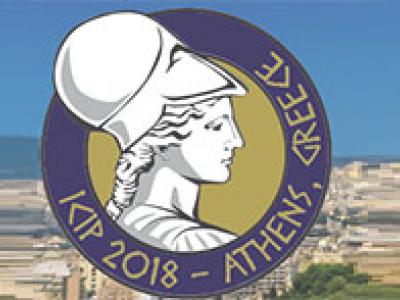
- Citation Author(s):
- Submitted by:
- Hang Duong
- Last updated:
- 4 October 2018 - 5:42am
- Document Type:
- Poster
- Document Year:
- 2018
- Event:
- Presenters:
- Manh-Quan Bui
- Paper Code:
- MP.P5.4
- Categories:
- Log in to post comments
In this work, we address human action recognition problem under viewpoint variation. The proposed model is formulated by wisely combining convolution neural network (CNN) model with principle component analysis (PCA). In this context, we pass real depth videos through a CNN model in a frame-wise manner. The view invariant features are extracted by employing convolution layers as mid-outputs and considered as 3D nonnegative tensors. The PCA algorithm is separately imposed on view-invariant high-level space of image and video groups to seek both local and holistic hidden dynamics information. To deal with noisy data and temporal misalignment, we utilize the Fourier temporal pyramid to encode temporal and obtain the final descriptors. Our proposed framework supplies a robust discriminative representation with low dimension and computational requirements. We evaluate the proposed method on two standard multiview depth video datasets. The experimental results show that our method has superior performance compared to other approaches.