Documents
Supplementary Materials
DiGS-3D: Diffusion Transformer as unstructured 3D Gaussian Splatting Generator - Supplementary Material
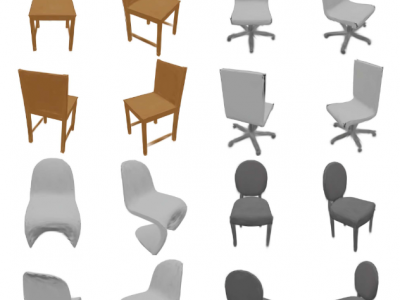
- DOI:
- 10.60864/yyhc-wb65
- Citation Author(s):
- Submitted by:
- - -
- Last updated:
- 10 February 2025 - 4:35am
- Document Type:
- Supplementary Materials
- Categories:
- Log in to post comments
Generation of 3D Gaussian Splatting (3D GS) representation suffers from the challenge of maintaining the visual aspect while preserving the geometry of generated objects. We introduce DiGS-3D, an efficient method for generation of 3D Gaussian Splatting representations generation using a diffusion transformer. While current 3D GS generative models use proxy representation as a way to structure the point cloud before processing, our method preserves the original 3D structure of the 3D GS representation. We use an encoder-only transformer architecture to model both the parameters of 3D gaussians and their relationships across the entire scene, and reach competitive metrics on the unconditional 3D GS generation task. We additionally introduce depth supervision for 3D radiance field generative diffusion model training, which we show improves the performance of our model.