Documents
Poster
DIRICHLET PROCESS MIXTURE MODELS FOR CLUSTERING I-VECTOR DATA
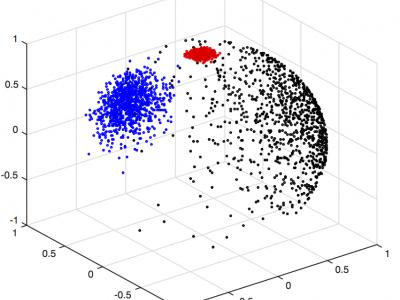
- Citation Author(s):
- Submitted by:
- Shreyas Seshadri
- Last updated:
- 28 February 2017 - 7:05am
- Document Type:
- Poster
- Document Year:
- 2017
- Event:
- Presenters:
- Shreyas Seshadri
- Paper Code:
- 1563
- Categories:
- Keywords:
- Log in to post comments
Non-parametric Bayesian methods have recently gained popularity in several research areas dealing with unsupervised learning. These models are capable of simultaneously learning the cluster models as well as their number based on properties of a dataset. The most commonly applied models are using Dirichlet process priors and Gaussian models, called as Dirichlet process Gaussian mixture models (DPGMMs). Recently, von Mises-Fisher mixture models (VMMs) have also been gaining popularity in modelling high-dimensional unit-normalized features such as text documents and gene expression data. VMMs are potentially more efficient in modeling certain speech representations such as i-vector data when compared to the GMM-based models, as they work with unit-normalized features based on cosine distance. The current work investigates the applicability of Dirichlet process VMMs (DPVMMs) for i-vector-based speaker clustering and verification, showing that they indeed show superior performance in comparison to DPGMMs in the tasks. In addition, we introduce an implementation of the DPVMMs with variational inference that is publicly available for use.