Documents
Presentation Slides
Presentation Slides
Discriminative Clustering with Cardinality Constraints
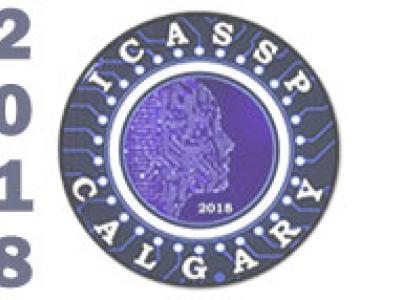
- Citation Author(s):
- Submitted by:
- Anh Pham
- Last updated:
- 25 April 2018 - 2:00pm
- Document Type:
- Presentation Slides
- Document Year:
- 2018
- Event:
- Presenters:
- Anh Pham
- Paper Code:
- 1817
- Categories:
- Log in to post comments
Clustering is widely used for exploratory data analysis in a variety of applications. Traditionally clustering is studied as an unsupervised task where no human inputs are provided. A recent trend in clustering is to leverage user provided side information to better infer the clustering structure in data. In this paper, we propose a probabilistic graphical model that allows user to provide as input the desired cluster sizes, namely the cardinality constraints. Our model also incorporates a flexible mechanism to inject control of the crispness of the clusters. Experiments on synthetic and real data demonstrate the effectiveness of the proposed method in learning with cardinality constraints in comparison with the current state-of-the-art.