Documents
Poster
DISCRIMINATIVE FEATURES FOR INCREMENTAL LEARNING CLASSIFIER
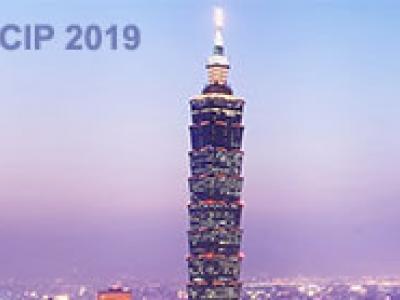
- Citation Author(s):
- Submitted by:
- Tin Lay Nwe
- Last updated:
- 20 September 2019 - 6:33am
- Document Type:
- Poster
- Document Year:
- 2019
- Event:
- Presenters:
- Tin Lay Nwe
- Paper Code:
- 2132
- Categories:
- Log in to post comments
An important problem in artificial intelligence is to develop an ef-
ficient system that can adapt to new knowledge in an incremen-
tal manner without forgetting previously learned knowledge. Al-
though Convolutional Neural Networks (CNNs) are good at learn-
ing strong classifier and discriminative features, CNNs can not per-
form well in incremental classifier learning due to the catastrophic
forgetting problem in the retraining process. In this paper, we pro-
pose a novel yet extremely simple approach to enhance the discrim-
inative property of features for incremental classifier learning. We
build a network for the universal feature space in which a group of
image classes have intra-class compactness and inter-class separa-
bility. And, we model each incremental class to have a maximum
margin from the rest of the models in universal space. Experiments
are conducted on CIFAR-100 dataset and IMage Database for Con-
text Aware Advertisement (IMDB-CAA) we collected. The results
demonstrate the superiority of our approach, improving performance
on CIFAR-100 dataset over state-of-the-art incremental learning sys-
tems. Furthermore, experiments on few-short incremental learning
setting show very promising performance although we use only 4%
of training samples on CIFAR-100 dataset.
Comments
DISCRIMINATIVE FEATURES FOR
DISCRIMINATIVE FEATURES FOR INCREMENTAL LEARNING CLASSIFIER
DISCRIMINATIVE FEATURES FOR
DISCRIMINATIVE FEATURES FOR INCREMENTAL LEARNING CLASSIFIER