Documents
Poster
DISCRIMINATIVE SALIENCY-POSE-ATTENTION COVARIANCE FOR ACTION RECOGNITION
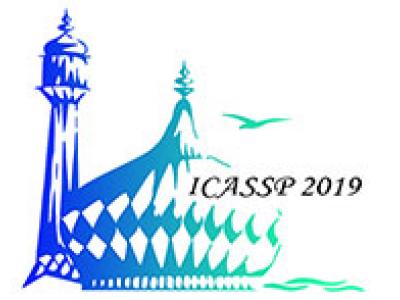
- Citation Author(s):
- Submitted by:
- Jianhai Zhang
- Last updated:
- 18 February 2019 - 6:34am
- Document Type:
- Poster
- Document Year:
- 2019
- Event:
- Presenters:
- Jianhai Zhang
- Paper Code:
- 3992
- Categories:
- Log in to post comments
Most covariance-based representations of actions are focused on the statistical features of poses by empirical averaging weighting. Note that these poses have a variety of saliency levels for different actions. Neglecting pose saliency could degrade the discriminative power of the covariance features, and further reduce the performance of action recognition. In this paper, we propose a novel saliency weighting covariance feature representation, Saliency-Pose-Attention Covariance(SPA-Cov), which reduces the negative effects from the ambiguous pose samples. Specifically, we utilize a discriminative approach to derive probability distribution of action categories for each pose, which is modeled by the uncertainty of information entropy to obtain the salient weighting. Experimental results show that our proposed method efficiently improves the discriminative power of the generated covariance. In some databases, the proposed SPA-Cov outperforms the state-of-the-art variant methods which are based on kernel matrix, Bayesian posterior features, temporal hierarchical features, etc.